- Basics of Time-Series Analysis
- Definition and Components
- Data Preparation
- Common Time-Series Models
- Moving Averages
- Autoregressive Integrated Moving Average (ARIMA)
- Exponential Smoothing State Space Models (ETS)
- Implementing Time-Series Analysis in Econometrics Homework
- Choosing the Right Model
- Estimation and Model Evaluation
- Diagnostic Checking
- Forecasting with Time-Series Models
- In-Sample and Out-of-Sample Forecasting
- Forecast Accuracy Metrics
- Practical Tips for Econometrics Homework
- Understand the Economic Context
- Documentation and Interpretation
- Seek Help When Needed
- Conclusion
Econometrics, situated at the intersection of economics and statistics, constitutes a pivotal branch that utilizes statistical methods for hypothesis testing and predicting future trends. At the core of econometrics lies time-series analysis, a fundamental component dedicated to scrutinizing data points gathered over a defined duration to discern patterns and facilitate predictions. The mastery of time-series analysis is indispensable for students embarking on academic pursuits in economics or related disciplines. This blog endeavors to demystify the intricacies of time-series analysis, offering a simplified guide tailored to equip students with the tools necessary to confidently approach their university assignments. By breaking down the complexities inherent in econometric tasks, this guide seeks to empower students, fostering a deeper understanding of the subject matter and enhancing their ability to navigate the challenges posed by time-series data. As the academic landscape continually evolves, the significance of time-series analysis in econometrics becomes increasingly pronounced, making it imperative for students to grasp its nuances. Through a comprehensive exploration of the basics, including the components of time-series data and the essential steps in data preparation, students can lay a solid foundation for more advanced analyses. Delving into common time-series models, such as moving averages, ARIMA, and ETS, further enriches students' analytical repertoire, providing them with a diverse toolkit to address a spectrum of real-world economic scenarios. The blog also underscores the practical implementation of time-series analysis in econometrics homework, emphasizing the importance of model selection, estimation, and evaluation. Insightful tips for forecasting, including in-sample and out-of-sample techniques, coupled with forecast accuracy metrics, equip students with the skills to translate theoretical knowledge into actionable insights. Whether you need assistance with your Econometrics homework or seek to deepen your understanding of time-series analysis, this guide offers invaluable support to enhance your learning journey.
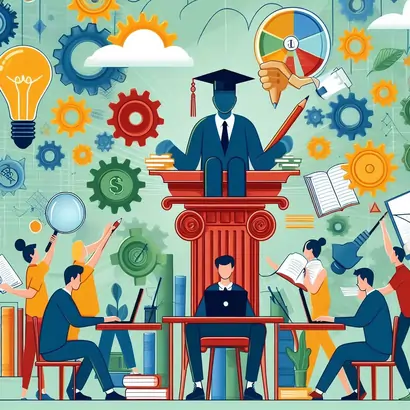
Moreover, the guide encourages students to bridge the gap between econometrics and real-world economics by contextualizing their analyses within broader economic theories and events. The emphasis on documentation, interpretation, and seeking assistance when needed serves as a practical roadmap for students navigating the intricacies of econometrics assignments. In conclusion, the blog aspires to serve as a beacon of clarity in the realm of time-series analysis for econometrics, acting as a valuable resource that propels students toward academic success. By unraveling the complexities and demystifying the jargon associated with econometrics, this guide aims to instill confidence in students, enabling them to not only excel in their university assignments but also to appreciate the practical implications of time-series analysis in shaping our understanding of economic phenomena and informing decision-making processes in the real world.
Basics of Time-Series Analysis
The fundamentals of time-series analysis constitute a cornerstone in the realm of econometrics, providing a foundational understanding for students venturing into the intricate world of analyzing temporal data. Central to this exploration is the comprehension of various components inherent in time-series data. The trend component, elucidating the long-term movement of data, sets the stage for discerning whether the observed phenomena are characterized by growth, decline, or stability. Simultaneously, the seasonal component sheds light on repetitive patterns influenced by external factors like seasons or holidays, offering valuable insights into periodic fluctuations. The cyclical component, extending its purview to medium to long-term undulations, serves as a vital lens through which economic or business cycles can be scrutinized. Amidst these identifiable components, the irregular component, often manifested as residuals, introduces an element of unpredictability, capturing random fluctuations not accounted for by the overarching trends or patterns. As students embark on mastering the basics of time-series analysis, meticulous data preparation emerges as a crucial step, necessitating the removal of outliers or errors and ensuring consistency in the frequency of data points. This foundational knowledge not only equips students with the skills to navigate through the complexities of econometric assignments but also lays the groundwork for more advanced analyses. With a solid understanding of the basics, students are better positioned to delve into the intricate models and methodologies that form the bedrock of time-series analysis in econometrics.
Definition and Components
Time-series analysis stands as a fundamental methodology in econometrics, involving the meticulous study of data collected over successive time intervals. In the context of econometrics, time-series data encompasses a diverse range of economic indicators, such as GDP, inflation rates, or stock prices. To navigate the intricacies of time-series analysis effectively, it is imperative to comprehend the distinct components inherent in this type of data.
The Trend Component embodies the overarching, long-term movement within the data, offering insights into whether the observed phenomenon is marked by an upward trajectory, a decline, or stability. Concurrently, the Seasonal Component unveils repeating patterns or fluctuations occurring within specific time frames, often influenced by external factors like seasons, holidays, or other recurring events. Delving into the medium to long-term undulations, the Cyclical Component captures fluctuations that are not necessarily repetitive, shedding light on economic or business cycles. The Irregular Component, represented by residuals, introduces an element of unpredictability, encapsulating random fluctuations that elude explanation from the trend, seasonal, or cyclical components.
Data Preparation
Before embarking on the intricate journey of analysis, students must ensure that their time-series data undergoes meticulous preparation to yield meaningful results. The process of Data Cleaning assumes paramount importance, requiring the removal of outliers or errors that could potentially distort the analysis and compromise the accuracy of results. Simultaneously, Frequency Adjustment demands attention to detail, necessitating the establishment of consistency in the time intervals between data points. This step ensures that the temporal aspect of the data is appropriately aligned, facilitating a more accurate representation of the underlying patterns. By laying a robust foundation through effective data preparation, students position themselves to engage in more nuanced and insightful time-series analysis, enhancing the rigor and validity of their econometric endeavors.
Common Time-Series Models
In the expansive landscape of time-series analysis within econometrics, the terrain is enriched by a repertoire of common models that empower students to distill meaningful insights from complex temporal data. A cornerstone in this collection of models is the Moving Averages model, which operates by smoothing fluctuations through the calculation of the average of a specified number of consecutive data points. This approach proves particularly valuable in discerning trends within a dataset. Another stalwart in the arsenal of time-series models is the Autoregressive Integrated Moving Average (ARIMA) model, a fusion of autoregressive (AR) and moving average (MA) components. ARIMA's efficacy lies in its ability to capture intricate patterns within time-series data, with careful consideration given to the order of differencing and lag terms. Complementing this, Exponential Smoothing State Space Models (ETS) offer a flexible framework encompassing error, trend, and seasonal components, accommodating diverse patterns present in economic data. As students navigate the intricacies of time-series analysis, these models serve as invaluable tools, each tailored to address specific nuances within the data. The discerning choice of a model hinges on an astute understanding of the data's characteristics, with considerations for trends, seasonality, and other discernible patterns. Mastery of these common time-series models not only equips students with the analytical prowess to unravel complex economic phenomena but also provides a solid foundation for tackling diverse real-world challenges. Through the assimilation of these models into their econometric toolkit, students are poised to unravel the dynamic nature of time-series data, unlocking the potential for more accurate predictions and a deeper understanding of the economic landscape.
Moving Averages
Moving Averages, a foundational concept in time-series analysis, emerges as a powerful tool to mitigate the impact of fluctuations within datasets. This technique achieves smoothness by calculating the average of a predetermined number of consecutive data points. The utility of moving averages lies prominently in its ability to discern and highlight underlying trends within the data, presenting an essential mechanism for identifying and understanding the directional movement of economic indicators. By effectively filtering out short-term noise, moving averages offer a clearer perspective, making them particularly useful for students seeking to unravel patterns and trends within time-series data for comprehensive econometric analyses.
Autoregressive Integrated Moving Average (ARIMA)
The Autoregressive Integrated Moving Average (ARIMA) model stands out as a sophisticated and widely employed tool in time-series analysis. A hybrid of autoregressive (AR) and moving average (MA) components, ARIMA excels in capturing intricate patterns within time-series data. Crucial to its application is a nuanced understanding of the order of differencing and lag terms, factors that significantly influence the model's performance. ARIMA's versatility makes it a preferred choice for students delving into complex time-series datasets, providing them with a robust framework to decipher the dynamic nature of economic phenomena and forecast future trends.
Exponential Smoothing State Space Models (ETS)
Exponential Smoothing State Space Models (ETS) represent a sophisticated class of models designed to address the intricacies of time-series data comprehensively. ETS models take into account the error, trend, and seasonality components, providing a flexible framework capable of capturing diverse patterns inherent in economic datasets. As students navigate the landscape of time-series analysis, ETS models offer a holistic approach, allowing for a nuanced exploration of data characteristics and the identification of underlying patterns. With their adaptability and comprehensive nature, ETS models stand as invaluable tools for students seeking to elevate their econometric analyses, offering a versatile means to interpret and forecast within the dynamic context of time-series data.
Implementing Time-Series Analysis in Econometrics Homework
The practical application of time-series analysis within the realm of econometrics forms a crucial phase for students translating theoretical knowledge into actionable insights within their academic assignments. A pivotal aspect of this implementation involves the judicious selection of the appropriate time-series model based on the unique characteristics of the data at hand. Whether it be the simplicity of Moving Averages, the sophistication of Autoregressive Integrated Moving Average (ARIMA), or the holistic approach of Exponential Smoothing State Space Models (ETS), students must discern which model aligns most effectively with the nuances of the given dataset. Estimation and model evaluation further underscore the implementation process, demanding the utilization of statistical software such as R, Python, or EViews to ensure accuracy and efficiency in the analysis. Techniques like the Akaike Information Criterion (AIC) or Bayesian Information Criterion (BIC) serve as guiding metrics for model selection, reinforcing the importance of a systematic approach in econometric analysis. Diagnostic checking becomes an indispensable step to validate the chosen model, assessing the randomness and normal distribution of residuals. As students venture into forecasting, distinguishing between in-sample and out-of-sample techniques becomes paramount, offering insights into predicting values within observed data and extending predictions beyond, respectively. Forecast accuracy metrics, including Mean Absolute Error (MAE) and Root Mean Squared Error (RMSE), become evaluative tools to gauge the precision of predictions. Through this implementation process, students not only hone their analytical skills but also gain a practical understanding of how time-series analysis contributes to unraveling economic patterns, thus bridging the theoretical and applied dimensions of econometrics within the context of their homework assignments.
Choosing the Right Model
The crux of effective time-series analysis lies in the astute selection of the appropriate model, a decision contingent upon the distinct characteristics of the data under scrutiny. As students embark on this crucial step, a nuanced consideration of the nature of the trend, seasonality, and other discernible patterns within the time series becomes imperative. By identifying these underlying features, students can align their choice of model with the inherent complexities of the data, laying the groundwork for a more accurate and insightful analysis. Whether addressing economic cycles, business trends, or seasonal variations, this step sets the stage for a comprehensive exploration of the data's temporal dynamics.
Estimation and Model Evaluation
The implementation of time-series analysis necessitates the use of sophisticated statistical software tools such as R, Python, or EViews for model estimation and subsequent evaluation. In this phase, techniques like the Akaike Information Criterion (AIC) or Bayesian Information Criterion (BIC) assume prominence, offering quantitative measures to aid in the selection of the most fitting model. These criteria serve as guiding metrics, providing students with a systematic approach to discerning the optimal balance between model complexity and goodness of fit. By leveraging these tools, students can navigate the intricate landscape of model selection, ensuring a robust foundation for their econometric analyses.
Diagnostic Checking
The journey of time-series analysis extends beyond model selection and estimation, delving into the realm of diagnostic checking to validate the chosen model's assumptions. Ensuring that the residuals are not only random but also follow a normal distribution is paramount. Diagnostic plots and statistical tests emerge as indispensable tools in this validation process, offering visual and numerical insights into the adequacy of the model. By rigorously assessing the randomness and distribution of residuals, students fortify the reliability of their chosen model, reinforcing the integrity of their econometric analyses and instilling a deeper understanding of the nuances inherent in time-series data.
Forecasting with Time-Series Models
Forecasting, the pinnacle of time-series analysis within econometrics, propels students into the realm of anticipating future trends and behaviors based on historical data patterns. In this pivotal phase, a dichotomy between in-sample and out-of-sample forecasting methods emerges as a foundational consideration. In-sample forecasting involves predicting values within the temporal span of the observed data, providing a close examination of the model's performance within the historical context. On the other hand, out-of-sample forecasting extends the predictive capabilities beyond the observed data, serving as a litmus test for the model's robustness and adaptability to unforeseen scenarios. As students navigate the intricacies of forecasting, the arsenal of forecast accuracy metrics, including Mean Absolute Error (MAE), Mean Squared Error (MSE), and Root Mean Squared Error (RMSE), becomes instrumental in gauging the precision and reliability of predictions. These metrics offer a quantitative assessment of the disparity between predicted and actual values, aiding students in fine-tuning their models for optimal performance. The implementation of forecasting techniques not only empowers students with the ability to make informed predictions but also cultivates a deeper understanding of the dynamic interplay between economic variables over time. By immersing themselves in the art and science of forecasting with time-series models, students bridge the theoretical underpinnings of econometrics with real-world implications, laying a foundation for sound decision-making in various economic spheres. Whether anticipating stock prices, inflation rates, or economic growth, the ability to forecast with precision transforms time-series analysis into a potent tool for students navigating the complexities of econometrics in their academic endeavors and beyond.
In-Sample and Out-of-Sample Forecasting
In the realm of time-series analysis, the dichotomy between in-sample and out-of-sample forecasting methods holds profound implications for students navigating the intricacies of econometrics. In-sample forecasting entails predicting values within the temporal confines of the observed data, offering a close examination of the model's efficacy within the historical context. Conversely, out-of-sample forecasting extends the predictive horizon beyond the confines of observed data, presenting a critical litmus test for the model's adaptability to unforeseen scenarios. As students delve into forecasting, comprehending the nuances and distinctions between these approaches becomes paramount, as it influences the robustness and applicability of their models in real-world scenarios. The ability to judiciously choose between in-sample and out-of-sample methods empowers students to make informed decisions and predictions, setting the stage for a more nuanced understanding of the dynamic nature of time-series data.
Forecast Accuracy Metrics
The evaluation of forecasting accuracy stands as an integral facet of time-series analysis, requiring students to employ metrics that gauge the effectiveness of their predictive models. Mean Absolute Error (MAE), Mean Squared Error (MSE), and Root Mean Squared Error (RMSE) emerge as essential metrics in this evaluative process. MAE quantifies the average magnitude of errors, providing insights into the model's precision. MSE takes it a step further by considering the squared errors, amplifying the impact of larger deviations. RMSE, an extension of MSE, offers a more interpretable metric by taking the square root of the average squared errors. As students grapple with these metrics, they gain a quantitative understanding of the disparity between predicted and actual values, refining their forecasting models for heightened accuracy. These accuracy metrics serve as benchmarks, guiding students in the continuous refinement of their time-series models, ultimately enhancing their ability to make reliable predictions and contributing to the broader field of econometrics.
Practical Tips for Econometrics Homework
Navigating the landscape of econometrics homework demands a strategic approach that extends beyond theoretical knowledge, emphasizing the practical application of time-series analysis. First and foremost, students are encouraged to contextualize their analyses within the broader economic landscape, linking the intricacies of time-series data to established economic theories and real-world events. This contextual understanding not only enhances the depth of analysis but also strengthens the bridge between theoretical concepts and practical implications. Documentation assumes paramount importance; each step of the analysis, from data preparation to model selection and forecasting, should be meticulously recorded. This not only serves as a guide for self-review but also facilitates effective communication of methodologies and findings. Interpretation of results within the specific economic problem or question posed is a crucial aspect, showcasing the student's ability to translate statistical insights into meaningful economic implications. Seeking assistance when faced with challenges is not a sign of weakness but a pragmatic step towards deeper understanding. Whether consulting professors, engaging with classmates, or leveraging online resources, seeking help fosters a collaborative learning environment. Additionally, embracing technological tools such as statistical software (e.g., R, Python, or EViews) enhances efficiency and precision in data analysis. Ultimately, students are reminded that econometrics is not merely an academic exercise but a gateway to applied research and policy analysis. By incorporating these practical tips into their econometrics homework approach, students not only conquer the immediate challenges of assignments but also cultivate a skill set that extends beyond the classroom, equipping them for success in the dynamic and data-driven world of economics.
Understand the Economic Context
In the realm of econometrics, understanding the economic context is paramount to deriving meaningful insights from time-series analysis. It is imperative for students to establish a link between their analyses and established economic theories or real-world events. This contextual understanding not only provides a more comprehensive perspective but also enriches the interpretation of results. By discerning how economic phenomena relate to the patterns identified in time-series data, students can offer more nuanced and insightful analyses, transcending mere statistical observations to grasp the economic implications of their findings.
Documentation and Interpretation
The meticulous documentation of each step in the analysis process is a fundamental practice that students should embrace in their econometrics homework. Clear documentation not only aids in self-review but also ensures transparency in methodologies employed. Equally important is the interpretation of findings, contextualizing them within the specific economic problem or question posed. This step requires students to delve beyond numerical results, providing a narrative that explains the economic significance of their analyses. By articulating the real-world implications of statistical outcomes, students not only showcase their analytical prowess but also reinforce the practical relevance of time-series analysis in understanding economic dynamics.
Seek Help When Needed
Recognizing the inherent challenges in econometrics and time-series analysis, students are encouraged to overcome obstacles by seeking help when needed. This proactive approach may involve consulting professors for clarification, engaging with classmates in collaborative learning, or tapping into online resources that offer additional explanations and examples. Embracing a willingness to seek assistance not only fosters a supportive learning environment but also reflects a commitment to understanding the complexities of econometrics. Through collaborative efforts and a willingness to learn from various sources, students can overcome challenges and enhance their proficiency in time-series analysis, ultimately contributing to a more enriching educational experience.
Conclusion
Time-series analysis is a powerful tool in econometrics, offering insights into economic trends and helping make informed predictions. This guide provides students with a simplified approach to tackle time-series analysis in their econometrics homework. By mastering the basics, understanding common models, and following practical tips, students can confidently apply time-series analysis to real-world economic problems. As they navigate their assignments, they'll gain valuable skills that extend beyond the classroom into the realms of economic research and policy analysis.
Similar Blogs
Welcome to the Economics Homework Helper blog! Here, you'll find insightful articles, tips, and resources to master your economics assignments. Stay updated with the latest trends, expert advice, and practical strategies to excel in your studies. Join our community and boost your academic success!