- Understanding the Basics of Maximum Likelihood Estimation
- What is Maximum Likelihood Estimation?
- The Likelihood Function
- Log-Likelihood Function
- The Steps Involved in Maximum Likelihood Estimation
- Formulating the Likelihood Function
- Maximizing the Likelihood Function
- Checking the Validity of Maximum Likelihood Estimates
- Applications of Maximum Likelihood Estimation
- Examples in Different Fields
- MLE in Regression Analysis
- Challenges and Common Pitfalls in Maximum Likelihood Estimation
- Sensitivity to Initial Values
- Assumptions of the Model
- Tips for Solving Maximum Likelihood Estimation Assignments
- Utilizing Statistical Software
- Seeking Assistance and Resources
- Conclusion
In the realm of statistics, Maximum Likelihood Estimation (MLE) emerges as a potent tool, wielding its influence across diverse fields to unravel the intricacies of estimating parameters within a statistical model. Particularly for students immersed in assignments centered on this subject, a profound grasp of the principles and methodologies underpinning MLE becomes paramount. Recognizing this educational need, the following paragraphs delve into the intricacies of Maximum Likelihood Estimation, serving as an indispensable guide for students navigating through the challenges of related assignments. At its core, MLE hinges on the pursuit of optimal parameter estimates that maximize the likelihood function—a fundamental concept that measures how well a statistical model aligns with observed data. Breaking this down into more digestible parts, the likelihood function, a linchpin of MLE, emerges as a pivotal construct, encapsulating the probability of observing given data under specific parameter values. The transition to the log-likelihood function, a common maneuver in MLE, simplifies calculations without altering the essence of the estimates. The journey through MLE involves a series of steps, commencing with the formulation of the likelihood function, tailored to the characteristics of the statistical model and observed data. If you need assistance with your econometrics homework, consider this guide as a valuable resource to enhance your understanding and proficiency in Maximum Likelihood Estimation and related concepts.
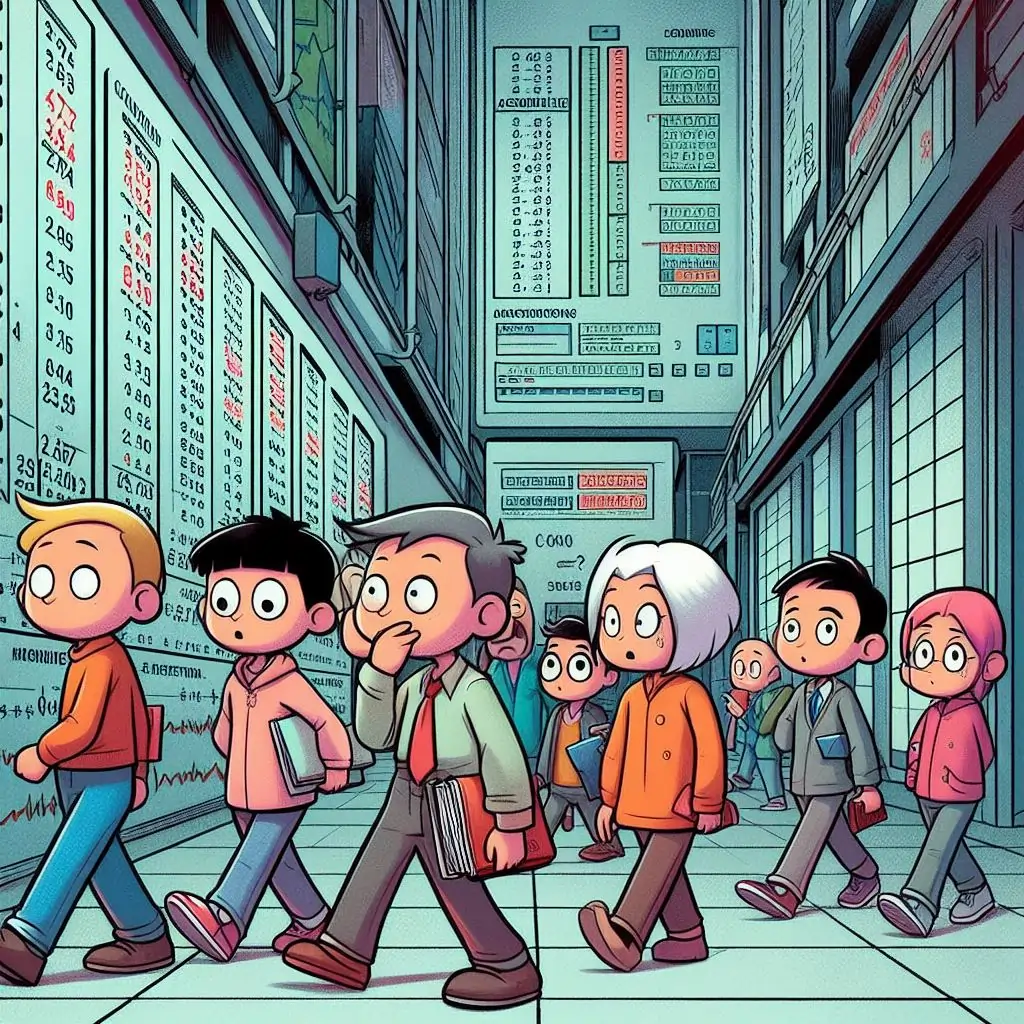
Subsequently, the heart of MLE lies in the maximization of this function, a process often involving calculus, derivative calculations, and the setting of equations to zero. Ensuring this optimization, scrutiny of the validity of Maximum Likelihood Estimates (MLE) is imperative. Methods like likelihood ratio tests and confidence intervals come into play, offering a lens through which students can evaluate the robustness and efficiency of their estimates. The practical utility of MLE extends across various domains, from economics and biology to finance and engineering. Real-world examples serve as invaluable illustrations, elucidating how MLE functions as a problem-solving mechanism in these disciplines. Amid the triumphs lie challenges, with sensitivity to initial values and assumptions of the model posing potential pitfalls. This demands a discerning approach from students, urging them to explore different starting points and critically assess the model's underlying assumptions. To fortify their efforts, students are encouraged to harness the power of statistical software such as R, Python, or MATLAB, which can streamline MLE calculations. Additionally, seeking guidance from professors, collaborating with peers, and tapping into online resources can prove invaluable in overcoming hurdles encountered during MLE assignments. In conclusion, Maximum Likelihood Estimation represents not just a statistical technique but a skill set vital for students navigating the complexities of parameter estimation. This blog aspires to serve as a compass, guiding students through the nuanced terrain of MLE, offering insights, practical examples, and strategies that empower them to approach assignments with confidence, competence, and a comprehensive understanding of this powerful statistical method.
Understanding the Basics of Maximum Likelihood Estimation
Understanding the basics of Maximum Likelihood Estimation (MLE) is foundational for delving into the intricacies of statistical parameter estimation. At its core, MLE is a powerful statistical technique employed to estimate the parameters of a given statistical model. The central tenet of MLE revolves around finding the values for these parameters that maximize the likelihood function, a pivotal concept measuring how well the model explains the observed data. To unravel the complexity of MLE for students, it is imperative to dissect the foundational components. Commencing with the definition of MLE, students must grasp the notion that it is not just a statistical method but a systematic approach to discerning the most likely values for the parameters of a model. The likelihood function, a key player in this pursuit, encapsulates the probability of observing the given data under specific parameter values. Transitioning further, the log-likelihood function often comes into play as a strategic tool, simplifying calculations without altering the ultimate goal of attaining maximum likelihood estimates. This foundational understanding sets the stage for students to comprehend the step-by-step process involved in MLE, which begins with the formulation of the likelihood function based on the statistical model and observed data. Moving forward, the crux of MLE lies in the maximization of this function, a process that typically involves mathematical optimization techniques such as taking derivatives, setting them to zero, and solving for the parameter values. Through this sequential exploration, students gain insight into the intricate dance between theory and application, laying the groundwork for their journey into the world of statistical estimation. A nuanced comprehension of these fundamental aspects not only equips students to navigate MLE assignments effectively but also provides a solid foundation for their broader understanding of statistical modeling and parameter estimation in diverse fields. Ultimately, this section acts as a gateway for students to embark on a more profound exploration of Maximum Likelihood Estimation, armed with the essential knowledge needed to tackle subsequent complexities in the realm of statistical analysis.
What is Maximum Likelihood Estimation?
Maximum Likelihood Estimation (MLE) stands as a statistical technique essential for estimating the parameters within a given statistical model. At its core, MLE operates on the fundamental principle of seeking parameter values that maximize the likelihood function. This function serves as a metric, measuring the extent to which the model explains the observed data. In essence, MLE endeavors to find the most probable values for the parameters that render the observed data most likely under the specified statistical model.
The Likelihood Function
The likelihood function emerges as a pivotal component in the landscape of Maximum Likelihood Estimation. It serves as a representation of the probability associated with observing the given data under specific parameter values. Despite its significance, students often grapple with comprehending the intricacies of the likelihood function. Breaking it down step by step becomes imperative to enhance accessibility, providing students with a clearer understanding of its role in the MLE process.
Log-Likelihood Function
In the realm of Maximum Likelihood Estimation, the log-likelihood function assumes a prominent role, offering a practical approach to simplify calculations and facilitate optimization. The log-likelihood is the natural logarithm of the likelihood function, a transformation that does not compromise the integrity of the maximum likelihood estimates. Rather, it renders the calculations more manageable, reducing complexity and aiding students in navigating the intricacies of MLE with greater ease and efficiency. This strategic utilization of the log-likelihood function exemplifies the synergy between mathematical optimization and statistical inference, highlighting the pragmatic aspects of MLE in the analytical landscape.
The Steps Involved in Maximum Likelihood Estimation
Embarking on the journey of Maximum Likelihood Estimation (MLE) necessitates a systematic approach, involving a series of key steps that unravel the complexities of parameter estimation within a statistical model. The initial step entails the formulation of the likelihood function, a critical task wherein students define the probability distribution describing the data and express it in terms of the model parameters. This foundational process sets the stage for the crux of MLE—maximizing the likelihood function. In this pursuit, students engage in mathematical optimization techniques, often involving calculus, where the derivatives are set to zero to solve for the parameter values that maximize the likelihood. As the optimization unfolds, the significance of this step becomes apparent, representing the essence of MLE as a method seeking the most plausible parameter values based on observed data. Following this optimization, a crucial phase ensues—checking the validity of the Maximum Likelihood Estimates (MLE). This involves employing statistical methods such as likelihood ratio tests and constructing confidence intervals to assess the reliability, consistency, and efficiency of the obtained estimates. This evaluative step ensures that the derived estimates align with the underlying statistical model and are robust representations of the observed data. In essence, the steps in Maximum Likelihood Estimation form a dynamic and interconnected process, guiding students through the intricate terrain of statistical inference and parameter estimation, ultimately empowering them to apply MLE effectively to real-world problems in diverse fields.
Formulating the Likelihood Function
The inception of Maximum Likelihood Estimation (MLE) demands a meticulous formulation of the likelihood function, marking the initial and pivotal step in this statistical journey. Here, students delve into the intricacies of defining the likelihood function, a task rooted in the characteristics of the statistical model and the observed data. This entails the specification of a probability distribution that aptly describes the dataset, artfully expressed in terms of the model parameters. The elegance of this formulation lies in its capacity to encapsulate the essence of the statistical relationship between the model parameters and the observed data, setting the foundation for subsequent MLE computations.
Maximizing the Likelihood Function
The heartbeat of Maximum Likelihood Estimation resonates in the pursuit of parameter values that maximize the likelihood function. This critical phase often immerses students in the world of calculus, as they grapple with derivatives, setting them to zero, and solving for the elusive parameter values that render the likelihood function at its zenith. While this step can be challenging for students, practical examples emerge as beacons of clarity, illuminating the path toward a comprehensive understanding of the optimization process within MLE. Through this mathematical orchestration, students unveil the essence of MLE as a method intricately woven with the artistry of statistical inference.
Checking the Validity of Maximum Likelihood Estimates
The triumphant culmination of MLE brings forth the obtained estimates, but the journey does not conclude without a critical evaluation of their validity. This assessment revolves around the scrutiny of consistency and efficiency, ensuring that the derived estimates align faithfully with the underlying statistical model and stand as robust representations of the observed data. Students embark on this evaluative phase armed with statistical tools such as likelihood ratio tests and confidence intervals, probing the quality and reliability of their Maximum Likelihood Estimates. This final checkpoint solidifies the integrity of the MLE process, affirming its prowess as a methodical and validated approach to parameter estimation in the realm of statistics.
Applications of Maximum Likelihood Estimation
The applications of Maximum Likelihood Estimation (MLE) span a diverse spectrum of fields, embodying its versatility as a powerful statistical tool. As students delve into the practical dimensions of MLE, they encounter its transformative impact on disciplines such as economics, biology, finance, and engineering. In economics, MLE assumes a pivotal role in estimating parameters for economic models, facilitating insights into phenomena ranging from market behavior to consumer preferences. The biological sciences leverage MLE to unravel the mysteries of genetic inheritance and population dynamics, enabling researchers to derive meaningful conclusions from observed data. Meanwhile, in the financial realm, MLE becomes a linchpin for risk assessment and portfolio optimization, providing a quantitative foundation for decision-making in investment strategies. Engineering applications of MLE extend to areas like signal processing and system identification, where accurate parameter estimation is paramount for optimizing performance. Real-world examples serve as compelling illustrations, showcasing how MLE functions as a problem-solving mechanism in these diverse disciplines. Through these applications, students not only witness the theoretical underpinnings of MLE in action but also gain a profound appreciation for its broad utility and impact on decision-making processes. The ubiquity of MLE across various fields underscores its significance as a unifying methodology, empowering students to recognize its potential applications and adapt its principles to address complex challenges in their academic and professional journeys. Thus, as students navigate the multifaceted landscape of MLE, they not only grasp its theoretical foundations but also glean insights into its transformative influence on diverse domains, cultivating a holistic understanding of its real-world relevance and implications.
Examples in Different Fields
The pervasive influence of Maximum Likelihood Estimation (MLE) extends across a myriad of fields, solidifying its status as a versatile and indispensable statistical methodology. Within the realm of economics, MLE serves as a cornerstone for parameter estimation in economic models, enabling the analysis of market trends, consumer behavior, and economic forecasting. In the biological sciences, MLE finds applications in genetic studies and population dynamics, providing a robust framework for extracting meaningful insights from observed biological data. Finance leverages MLE for risk assessment, portfolio optimization, and modeling financial phenomena, contributing to informed decision-making in investment strategies. Engineering domains, including signal processing and system identification, benefit from MLE's precision in estimating parameters critical for optimizing the performance of complex systems. As students navigate the intricacies of MLE, exploring real-world examples in these diverse fields becomes instrumental, offering tangible illustrations of its applicability and reinforcing the practical significance of mastering this statistical methodology.
MLE in Regression Analysis
In the specific context of regression analysis, Maximum Likelihood Estimation assumes a pivotal role, guiding the estimation of parameters within regression models. Regression analysis, a foundational statistical technique, explores relationships between variables, and MLE enhances its precision by determining the parameter values that maximize the likelihood of observing the given data. Students delving into MLE in the context of regression gain a nuanced understanding of how this method contributes to the accurate estimation of coefficients, intercepts, and other model parameters. This comprehension empowers students to apply MLE confidently to practical scenarios within their assignments, bridging the gap between theoretical knowledge and its pragmatic application in statistical analysis and modeling. As students grapple with regression-related assignments, a solid grasp of MLE becomes not only a theoretical asset but a practical tool for unraveling relationships and making informed predictions in diverse fields.
Challenges and Common Pitfalls in Maximum Likelihood Estimation
Navigating the terrain of Maximum Likelihood Estimation (MLE) is not without its challenges, as students and practitioners encounter nuanced intricacies that demand a discerning approach. One prominent challenge lies in the sensitivity of MLE to initial values. The optimization process can be influenced by the starting points chosen for parameter values, potentially leading to convergence issues or suboptimal solutions. This underscores the importance of exploring multiple starting points to enhance the robustness of the estimation process. Another critical consideration involves the assumptions inherent in the statistical model employed for MLE. Ensuring that these assumptions hold true in the given context is paramount for the validity of estimates. Students must critically assess the model's assumptions, recognizing that deviations may compromise the reliability of results. As the MLE journey unfolds, practitioners may encounter the common pitfall of overfitting, wherein the model becomes excessively complex, capturing noise in the data rather than underlying patterns. This highlights the delicate balance required in model selection, emphasizing the need for parsimony and a thorough understanding of the underlying data structure. Additionally, MLE's reliance on asymptotic properties for inference poses challenges in scenarios with limited data, necessitating caution in concluding when sample sizes are small. Acknowledging these challenges and pitfalls within the MLE framework equips students and practitioners with a heightened awareness, encouraging a thoughtful and iterative approach to parameter estimation. It underscores the importance of robustness checks, model diagnostics, and a critical evaluation of assumptions, ultimately fostering a more resilient and reliable application of Maximum Likelihood Estimation in the face of real-world complexities.
Sensitivity to Initial Values
In the realm of Maximum Likelihood Estimation (MLE), a critical challenge emerges in the form of sensitivity to initial parameter values. Students embarking on MLE endeavors must recognize that the optimization process can be highly contingent on the starting points chosen for parameter values. The sensitivity to these initial values can lead to convergence issues or, in some cases, suboptimal solutions. To navigate this challenge effectively, students are advised to explore different starting points systematically. By doing so, they gain insights into the stability of the estimation process and mitigate the risk of converging to local maxima rather than the global maximum. This sensitivity underscores the importance of a robust and iterative approach to parameter estimation within the MLE framework.
Assumptions of the Model
The efficacy of Maximum Likelihood Estimation (MLE) hinges on the adherence to specific assumptions about the underlying statistical model. These assumptions serve as the bedrock upon which the estimation process relies, and students must engage in a critical assessment to ascertain their validity in the context of their assignments. Whether it be assumptions regarding the distribution of the data, independence of observations, or other model-specific conditions, a meticulous evaluation is essential. Deviations from these assumptions can compromise the integrity of MLE estimates, emphasizing the importance of a nuanced understanding of the underlying model structure. Students navigating MLE assignments are encouraged to adopt a vigilant stance, recognizing that the validity of their estimates is contingent on the fidelity with which these assumptions hold in the given context. This critical evaluation not only safeguards the reliability of MLE results but also fosters a deeper comprehension of the interplay between statistical models and real-world data, enhancing the overall rigor of the estimation process.
Tips for Solving Maximum Likelihood Estimation Assignments
Mastering Maximum Likelihood Estimation (MLE) assignments requires a strategic and methodical approach, blending theoretical understanding with practical application. First and foremost, students are encouraged to leverage statistical software such as R, Python, or MATLAB. These tools streamline the computational aspects of MLE, allowing for efficient parameter estimation and optimization. Familiarity with these software platforms not only enhances computational efficiency but also provides students with valuable skills applicable in various analytical contexts. Additionally, seeking assistance and resources proves invaluable in navigating the intricacies of MLE. Whether through engaging with professors, collaborating with peers, or tapping into online tutorials and forums, students can gain insights, clarification, and diverse perspectives that enrich their understanding. Furthermore, a deep dive into practical examples and case studies offers a tangible connection between theoretical concepts and real-world applications. By applying MLE to concrete problems, students can solidify their comprehension and develop problem-solving skills that transcend the confines of textbook scenarios. Emphasizing the importance of thorough model diagnostics and sensitivity analyses, students should critically evaluate the assumptions underpinning their MLE models. Robustness checks, such as likelihood ratio tests and cross-validation, can fortify the validity of estimates and enhance the overall reliability of the MLE process. Lastly, time management plays a pivotal role in successful MLE assignment completion. Breaking down complex problems into manageable steps, allocating sufficient time for each component, and maintaining a disciplined work schedule contribute to a more systematic and stress-free approach. By integrating these tips, students can not only unravel the intricacies of Maximum Likelihood Estimation but also cultivate a holistic and practical skill set essential for their academic and professional journeys in statistical analysis.
Utilizing Statistical Software
In the dynamic landscape of Maximum Likelihood Estimation (MLE), the adept use of statistical software emerges as a crucial asset for students navigating complex assignments. Platforms like R, Python, and MATLAB offer robust environments for performing MLE calculations with efficiency and precision. Leveraging these tools can significantly streamline the assignment-solving process, allowing students to focus on the conceptual intricacies of MLE rather than getting entangled in manual computations. Familiarity with statistical software not only enhances computational proficiency but also equips students with practical skills transferrable to various analytical tasks. This proficiency in utilizing software platforms becomes particularly relevant as datasets and models grow in complexity, emphasizing the importance of integrating technology seamlessly into the MLE workflow.
Seeking Assistance and Resources
The path to mastering Maximum Likelihood Estimation is often paved with challenges, and students are encouraged to proactively seek assistance when needed. Professors, classmates, and online resources serve as invaluable pillars of support, offering insights and clarifications that can illuminate the intricacies of MLE. Engaging with professors provides an opportunity for personalized guidance, clarifying doubts, and fostering a deeper understanding of theoretical concepts. Collaborating with peers encourages knowledge-sharing and peer learning, while online resources, including textbooks, tutorials, and forums dedicated to MLE, offer a wealth of supplementary materials. These resources provide diverse perspectives, alternative explanations, and practical examples that augment the learning experience. By cultivating a proactive approach to seeking assistance and leveraging a spectrum of resources, students can navigate challenges in understanding and implementing MLE with resilience and a well-rounded understanding of this powerful statistical methodology.
Conclusion
Maximum Likelihood Estimation (MLE) may appear formidable initially, but with a systematic approach and practical examples, students can grasp its principles. This blog demystifies MLE, providing a step-by-step guide, diverse field applications, and strategies to overcome common challenges. The assignment solver's approach equips students with the confidence and accuracy needed to navigate MLE assignments successfully.