- Understanding Longitudinal Data Analysis:
- Capturing Change:
- Controlling for Individual Heterogeneity:
- Enhancing Statistical Power:
- Applications in Economics
- Labor Economics:
- Health Economics:
- Development Economics:
- Key Concepts in Longitudinal Data Analysis:
- Panel Data Structures:
- Fixed Effects vs. Random Effects Models:
- Time-series Econometrics:
- Dynamic Panel Models:
- Strategies for Tackling Econometrics Homework:
- Case Study: Analyzing Wage Dynamics Using Panel Data>
- Conclusion
In the realm of economics, understanding longitudinal data analysis is paramount, as it involves observing the same subjects repeatedly over time, a method commonly referred to as panel data. Mastering this analytical technique is crucial for economists as it allows them to analyze trends, patterns, and relationships within data that evolve over time, providing invaluable insights into dynamic processes within various economic phenomena. For students embarking on Econometrics homework, a solid grasp of longitudinal data analysis is essential for success, serving as the cornerstone for rigorous empirical analysis. In this comprehensive guide, we will delve into the fundamentals of longitudinal data analysis, exploring its applications in economics across diverse fields such as labor economics, health economics, development economics, and finance. By understanding the nuances of panel data structures, the distinctions between fixed effects and random effects models, and the intricacies of time-series econometrics, students can effectively analyze longitudinal data and derive meaningful conclusions. Furthermore, we will discuss strategies to tackle Econometrics homework with confidence, emphasizing the importance of data preparation, model selection, estimation techniques, interpretation of results, and robustness checks. Through practical examples and case studies, students will learn how to apply econometric methods to real-world data, enhancing their analytical skills and critical thinking abilities. By mastering longitudinal data analysis, students will not only excel in their academic pursuits but also be well-equipped to address complex economic questions and contribute meaningfully to the field of economics. This guide can provide valuable insights and strategies to help you succeed in your Econometrics homework.
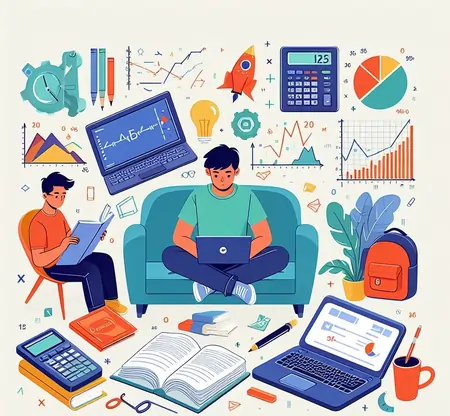
Whether examining wage dynamics, healthcare outcomes, financial market dynamics, or policy impacts, longitudinal data analysis provides a powerful framework for understanding and interpreting the dynamics of economic phenomena over time. With dedication, practice, and a solid understanding of econometric principles, students can navigate the complexities of longitudinal data analysis with confidence, laying the groundwork for successful careers in economics and related fields. In conclusion, longitudinal data analysis is a cornerstone of modern econometrics, offering a rich and versatile toolkit for empirical research in economics. By mastering this analytical technique, students can unlock new insights into economic phenomena, enriching our understanding of the world around us and paving the way for future advancements in economic theory and policy.
Understanding Longitudinal Data Analysis:
Understanding longitudinal data analysis is essential for economists and researchers aiming to explore changes in variables over time. Unlike cross-sectional data, which provides a static snapshot of a population at a single moment, longitudinal data tracks the trajectories of individuals, firms, or other entities across multiple time periods, offering a dynamic perspective on phenomena. This longitudinal approach offers several advantages, including the ability to capture temporal trends, identify patterns of change, and assess the impact of interventions or policies over time. By following subjects over time, researchers can also control for individual-level heterogeneity, leading to more accurate and robust estimates. Moreover, longitudinal data often yield larger sample sizes compared to cross-sectional data, enhancing statistical power and enabling more precise analyses. This longitudinal perspective is particularly valuable in fields such as labor economics, health economics, and development economics, where understanding the dynamics of change over time is crucial for informing policy decisions and interventions. Overall, longitudinal data analysis provides a powerful framework for investigating complex phenomena and uncovering insights into the dynamics of change, offering researchers a valuable tool for advancing knowledge and addressing real-world challenges in economics and beyond.
Capturing Change:
Longitudinal data analysis is instrumental in capturing the dynamic changes that occur within individuals or entities over time, providing economists with invaluable insights into the evolution of various economic processes. Unlike cross-sectional data, which offers only a static snapshot of a population at a single point in time, longitudinal data tracks the trajectories of subjects over multiple time periods, allowing researchers to observe and analyze trends, patterns, and fluctuations. This longitudinal perspective enables economists to understand how variables change over time, identify critical turning points, and uncover underlying dynamics that may not be apparent in cross-sectional analyses alone. By capturing change, longitudinal data analysis empowers economists to gain a deeper understanding of complex economic phenomena and formulate more effective strategies for addressing them.
Controlling for Individual Heterogeneity:
One of the significant advantages of longitudinal data analysis is its ability to control for individual-level differences or heterogeneity that may confound analyses in cross-sectional studies. By following the same subjects over time, researchers can account for factors such as innate abilities, preferences, or behaviors that vary between individuals and may influence the outcomes of interest. This longitudinal approach allows economists to isolate the effects of specific variables of interest while holding constant individual-specific factors, thereby enhancing the internal validity of their analyses. By controlling for individual heterogeneity, longitudinal data analysis enables researchers to make more precise and reliable estimates of the relationships between variables, reducing the risk of spurious correlations or biased results.
Enhancing Statistical Power:
Longitudinal data sets often encompass larger sample sizes compared to cross-sectional data, thereby enhancing the statistical power of analyses and enabling more precise estimates of parameters. With a larger number of observations available for analysis, economists can detect smaller effects with greater confidence, increasing the reliability and robustness of their findings. This enhanced statistical power allows researchers to conduct more rigorous hypothesis tests, identify subtle patterns or relationships within the data, and draw more accurate conclusions about the phenomena under study. Moreover, the larger sample sizes provided by longitudinal data sets enable economists to explore heterogeneity across different subgroups or populations more effectively, facilitating more nuanced and comprehensive analyses of economic phenomena. By enhancing statistical power, longitudinal data analysis equips economists with the tools they need to uncover meaningful insights and contribute to the advancement of economic knowledge.
Applications in Economics
Longitudinal data analysis finds widespread applications in various economic fields, playing a crucial role in advancing our understanding of dynamic economic processes and informing policy decisions. In labor economics, longitudinal data analysis allows researchers to study wage dynamics, employment patterns, and career trajectories over time, providing insights into factors influencing labor market outcomes and informing labor market policies. Health economics benefits from longitudinal data by enabling the analysis of healthcare interventions, disease progression, and health outcomes over time, aiding policymakers in designing effective healthcare policies and interventions. Development economics utilizes longitudinal data to investigate the long-term effects of policies and programs on economic development, poverty alleviation, and social mobility, guiding policymakers in crafting sustainable development strategies. In finance, longitudinal data analysis is essential for examining stock price movements, financial market dynamics, and portfolio performance over time, contributing to our understanding of asset pricing, risk management, and financial market efficiency. Moreover, longitudinal data analysis is valuable in studying consumer behavior, market dynamics, and firm performance, providing insights into market trends, competition dynamics, and business strategies. By leveraging longitudinal data, economists can address complex economic questions, test theoretical models, and evaluate the effectiveness of policy interventions, ultimately contributing to evidence-based policymaking and the advancement of economic theory. With the increasing availability of longitudinal data sources and advancements in econometric techniques, the applications of longitudinal data analysis in economics are likely to continue expanding, offering new opportunities for empirical research and policy analysis in diverse economic domains.
Labor Economics:
Longitudinal data analysis plays a pivotal role in labor economics, enabling researchers to delve into wage dynamics, employment patterns, and career trajectories over time. By tracking individuals or cohorts across multiple time periods, economists can analyze how wages change over the course of a career, identifying factors such as education, experience, and job tenure that influence earnings growth. Moreover, longitudinal data facilitates the examination of employment transitions, such as job changes, layoffs, or retirements, shedding light on labor market dynamics and the resilience of individuals in response to economic shocks. Understanding wage dynamics and employment patterns is essential for policymakers seeking to design effective labor market policies, such as minimum wage laws, training programs, and unemployment benefits, to promote economic opportunity and social mobility.
Health Economics:
Longitudinal data analysis is instrumental in health economics, where researchers seek to understand the impact of healthcare interventions, disease progression, and health outcomes over time. By following individuals or patient cohorts longitudinally, economists can evaluate the effectiveness of medical treatments, preventive interventions, and public health policies in improving health outcomes and reducing healthcare costs. Longitudinal studies also enable researchers to study disease trajectories, risk factors, and comorbidities, providing insights into the natural history of diseases and informing strategies for disease prevention and management. Moreover, longitudinal data allows economists to assess the long-term economic consequences of health shocks, such as disability, chronic illness, or premature mortality, on individuals, families, and healthcare systems, highlighting the importance of investing in preventive care and early intervention programs to mitigate the economic burden of disease.
Development Economics:
Longitudinal data analysis plays a crucial role in development economics, where researchers aim to understand the long-term effects of policies and programs on economic development and poverty alleviation. By tracking individuals, households, or communities over time, economists can assess the impact of interventions such as education programs, microfinance initiatives, and infrastructure projects on income growth, asset accumulation, and poverty reduction. Longitudinal studies also enable researchers to study intergenerational mobility, social capital formation, and the transmission of poverty across generations, providing insights into the drivers of persistent poverty and inequality. Moreover, longitudinal data allows economists to evaluate the sustainability of development interventions and identify strategies for promoting inclusive and equitable growth, ultimately contributing to evidence-based policymaking and the design of effective poverty reduction strategies.
Key Concepts in Longitudinal Data Analysis:
Before delving into econometrics homework, students must grasp fundamental concepts in longitudinal data analysis to effectively analyze and interpret data over time. One critical concept is understanding panel data structures, which involve observing the same subjects repeatedly over multiple time periods. Panel data can be categorized as balanced, where the number of observations for each subject is consistent across time periods, or unbalanced, where the number of observations may vary. Additionally, students should comprehend the distinction between fixed effects and random effects models. Fixed effects models control for time-invariant individual heterogeneity by including dummy variables for each individual in the analysis, whereas random effects models assume that individual effects are uncorrelated with the regressors. Understanding when to apply each model type is crucial for obtaining unbiased and efficient estimates. Time-series econometrics is another essential concept in longitudinal data analysis, as it involves analyzing temporal dependencies and autocorrelation within the data. Students must familiarize themselves with techniques such as autoregressive models, moving averages, and time-series regression analysis to account for time-varying factors and dynamic relationships. Furthermore, students should learn about dynamic panel models, which extend static panel models by incorporating lagged dependent variables and capturing dynamic relationships over time. Dynamic panel models are particularly useful for analyzing economic processes with inertia or persistence, such as investment decisions or consumption behavior. By mastering these key concepts in longitudinal data analysis, students can approach econometrics homework with confidence, effectively applying appropriate techniques to analyze and interpret longitudinal data and derive meaningful insights into economic phenomena.
Panel Data Structures:
Panel data, also known as longitudinal data, are structured to observe the same entities over multiple time periods. Understanding the structure of panel data is essential for economists analyzing longitudinal data. Panels can be categorized as balanced or unbalanced, depending on whether the number of observations for each entity remains constant across time periods. Moreover, panel data consist of individual and time dimensions, where individual units (such as individuals, firms, or countries) are observed over time, allowing for the examination of changes within entities over time. By comprehending panel data structures, economists can appropriately analyze and interpret longitudinal data, accounting for individual-specific and time-specific effects.
Fixed Effects vs. Random Effects Models:
In panel data analysis, economists often employ fixed effects and random effects models to account for individual-level heterogeneity. Fixed effects models include dummy variables for each individual in the analysis, controlling for time-invariant individual characteristics. On the other hand, random effects models assume that individual effects are uncorrelated with the regressors, allowing for more efficient estimation when individual effects are random and unobserved. Understanding the differences between fixed effects and random effects models is crucial for choosing the appropriate model specification and obtaining unbiased estimates in panel data analysis.
Time-series Econometrics:
Time-series econometrics involves applying statistical techniques to analyze temporal dependencies and autocorrelation within longitudinal data. By examining the time-series properties of data, economists can identify trends, seasonality, and cyclical patterns, facilitating the modeling and forecasting of economic variables over time. Time-series techniques include autoregressive integrated moving average (ARIMA) models, vector autoregression (VAR) models, and spectral analysis, among others. Mastery of time-series econometrics enables economists to effectively model and interpret longitudinal data, capturing the dynamic nature of economic phenomena and making informed policy recommendations.
Dynamic Panel Models:
Dynamic panel models extend static panel models by incorporating lagged dependent variables and capturing dynamic relationships over time. These models are particularly useful for analyzing economic processes with inertia or persistence, such as investment decisions, consumption behavior, or technological diffusion. By accounting for lagged effects and dynamic relationships, dynamic panel models provide more accurate estimates of parameters and enhance the understanding of temporal dynamics in longitudinal data. Mastery of dynamic panel models equips economists with the tools to investigate complex economic phenomena and derive meaningful insights into the dynamics of change over time.
Strategies for Tackling Econometrics Homework:
Tackling econometrics homework requires careful planning, attention to detail, and a solid understanding of both theoretical concepts and practical applications. One crucial strategy is to thoroughly understand the homework requirements by carefully reading the prompt and identifying the specific questions or tasks assigned. This initial step ensures that students have a clear understanding of what is expected and can effectively plan their approach to the homework. Additionally, data preparation is essential for conducting meaningful analyses. Students should clean and prepare the data for analysis, addressing issues such as missing values, outliers, and data discrepancies to ensure the reliability and validity of their results. Once the data is prepared, selecting an appropriate econometric model is crucial. Students should consider factors such as the nature of the data, the research question, and the assumptions underlying different model specifications. Whether choosing between fixed effects and random effects models in panel data analysis or selecting between different time-series techniques, students must carefully evaluate the strengths and limitations of each approach. Furthermore, effective estimation and inference are essential for drawing valid conclusions from econometric analyses. Students should accurately estimate the chosen models using appropriate statistical software and conduct hypothesis tests, calculate standard errors, and assess model fit and significance. Interpreting the results of the analysis is another critical step in tackling econometrics homework. Students should discuss the economic implications of their findings, critically evaluate the robustness of their results, and consider any limitations or caveats in their analysis. Finally, seeking feedback from peers or instructors and incorporating constructive criticism can help students refine their analyses and improve their understanding of econometric principles. By following these strategies, students can approach econometrics homework with confidence, effectively applying theoretical concepts to real-world data and deriving meaningful insights into economic phenomena.
- Understand the Homework Requirements: Start by thoroughly reading the homework prompt to grasp the specific questions or tasks assigned. Take note of any provided data and the required econometric techniques.
- Data Preparation: Clean and prepare the data meticulously for analysis, ensuring consistency, completeness, and proper formatting. Address issues such as missing values, outliers, and data discrepancies to maintain data integrity.
- Model Selection: Choose suitable econometric models based on the data's nature and the research question. Consider factors like endogeneity, serial correlation, and heteroscedasticity to ensure model appropriateness.
- Estimation and Inference: Utilize appropriate statistical software like R, Stata, or Python to estimate selected models. Perform hypothesis tests, calculate standard errors, and assess model fit and significance.
- Interpretation and Reporting: Interpret analysis results within the research question's context. Discuss the economic implications of findings and acknowledge any analysis limitations.
- Sensitivity Analysis: Conduct sensitivity analysis to gauge result robustness under varied model specifications, sample sizes, or estimation techniques, enhancing result reliability.
- Peer Review: Solicit feedback from peers or instructors to review your analysis and interpretations. Incorporate constructive criticism to refine your work and strengthen analysis validity.
Case Study: Analyzing Wage Dynamics Using Panel Data>
To exemplify the utilization of longitudinal data analysis in economics homework, let's delve into a case study on wage dynamics employing panel data. Imagine we possess panel data encompassing individual wages, educational attainment, and work experience for a cohort of workers across several years. In this scenario, longitudinal data analysis enables us to scrutinize how wages evolve over time in response to varying levels of education and experience. By tracking the same individuals over multiple years, we can discern patterns, trends, and factors influencing wage growth. This case study underscores the significance of longitudinal data in unraveling the intricacies of labor market dynamics, informing policies related to education, training, and workforce development, and elucidating the drivers of income disparities. Through rigorous econometric analysis, researchers can derive insights into wage determination, labor market trends, and the interplay between human capital accumulation and earnings trajectories, contributing to a deeper understanding of economic phenomena and guiding evidence-based policymaking.
- Data Description: The panel dataset comprises data on wages, education, work experience, and additional demographic variables for a cohort of individuals observed over a decade. This rich dataset enables a comprehensive examination of wage dynamics and their determinants overtime, offering insights into the evolving labor market landscape.
- Research Question: The primary research objective is to explore the factors influencing wage growth over time. Specifically, we aim to investigate the effects of education, work experience, and other relevant factors on the rate of wage increase. By analyzing these determinants, we can better understand the drivers of wage dynamics and inform policies aimed at promoting economic opportunity and income growth.
- Econometric Model: To address our research question, we will estimate a fixed effects panel model to control for individual-specific heterogeneity. This model specification includes individual characteristics, lagged dependent variables, and time dummies to capture time trends accurately. By controlling for individual effects and time trends, we can obtain unbiased estimates of the relationships between variables.
- Results and Interpretation: After estimating the econometric model, we will analyze the regression results to assess the impact of education, work experience, and other covariates on wage growth. We will interpret the findings within the context of the research question, discussing their economic implications and potential policy recommendations to promote wage growth and socioeconomic mobility.
- Robustness Checks: To ensure the reliability of our results, we will conduct sensitivity analysis by estimating alternative model specifications, such as random effects models or dynamic panel models. This robustness check allows us to test the stability of our findings under different modeling assumptions and ascertain the robustness of our conclusions. By conducting rigorous robustness checks, we can enhance the credibility and validity of our econometric analysis.
Conclusion
Longitudinal data analysis is a powerful tool for economists to study dynamic processes and relationships over time. Mastering this analytical technique is essential for students tackling econometrics homework in university courses. By understanding the key concepts, applying appropriate econometric models, and interpreting the results effectively, students can navigate through complex longitudinal data and derive meaningful insights for economic analysis. With practice and perseverance, students can enhance their skills in longitudinal data analysis and excel in econometrics homework, laying a solid foundation for future research and professional endeavors in economics.
Similar Blogs
Welcome to the Economics Homework Helper blog! Delve into our comprehensive collection of articles that explore economic theories, trends, and practical applications. Whether you're a student looking to strengthen your understanding or a professional seeking insightful analysis, our blog provides valuable resources to enhance your knowledge. Stay informed and inspired with our expertly curated content.