- Understanding Hypothesis Testing in Econometrics
- Formulating Hypotheses
- Null and Alternative Hypotheses
- Choosing the Significance Level
- Selecting a Test Statistic
- Collecting and Analyzing Data
- Interpreting Results
- Practical Application in University Assignments
- Selecting Relevant Topics
- Defining Variables and Hypotheses
- Data Collection and Preparation
- Choosing the Right Test Statistic
- Conducting the Hypothesis Test
- Interpreting Results and Drawing Conclusions
- Conclusion
Econometrics, as a specialized branch of economics, serves as the intersection between economic theory and statistical methods, facilitating the testing of hypotheses and the prediction of future trends. At its core, econometric analysis relies heavily on the crucial tool of hypothesis testing, an integral process empowering both seasoned researchers and aspiring students to extract meaningful conclusions from raw data. This proficiency in hypothesis testing is a cornerstone for making informed decisions and accurate predictions, forming the bedrock of economic research and analysis. As we embark on an exploration of the significance of hypothesis testing in econometrics, it becomes evident that this methodological approach is not merely an academic exercise but a practical and essential skill set for anyone involved in economic inquiries. For students navigating the labyrinth of university assignments, understanding and effectively utilizing hypothesis testing can be the key to unlocking the analytical potential required to excel in econometrics coursework. By grasping the intricacies of formulating hypotheses, discerning between null and alternative hypotheses, and judiciously choosing the significance level, students lay a solid foundation for robust econometric analyses. Selecting an appropriate test statistic, be it a t-test, F-test, or chi-square test, becomes the linchpin in the hypothesis testing process, demanding a keen understanding of the data at hand and the specific economic relationship under scrutiny. Armed with the proficiency to collect, clean, and prepare relevant data, students can seamlessly transition into the realm of conducting hypothesis tests using sophisticated econometric software, transforming theoretical concepts into tangible results. The interpretation of these results is the final frontier, where students showcase not only their statistical acumen but also their ability to contextualize findings within the broader economic landscape. In the crucible of university assignments, the practical application of hypothesis testing becomes a proving ground for students, allowing them to demonstrate their mastery over econometric principles. If you need assistance with your Econometrics homework, understanding and effectively applying hypothesis testing principles are essential for tackling assignments and conducting robust econometric analyses.
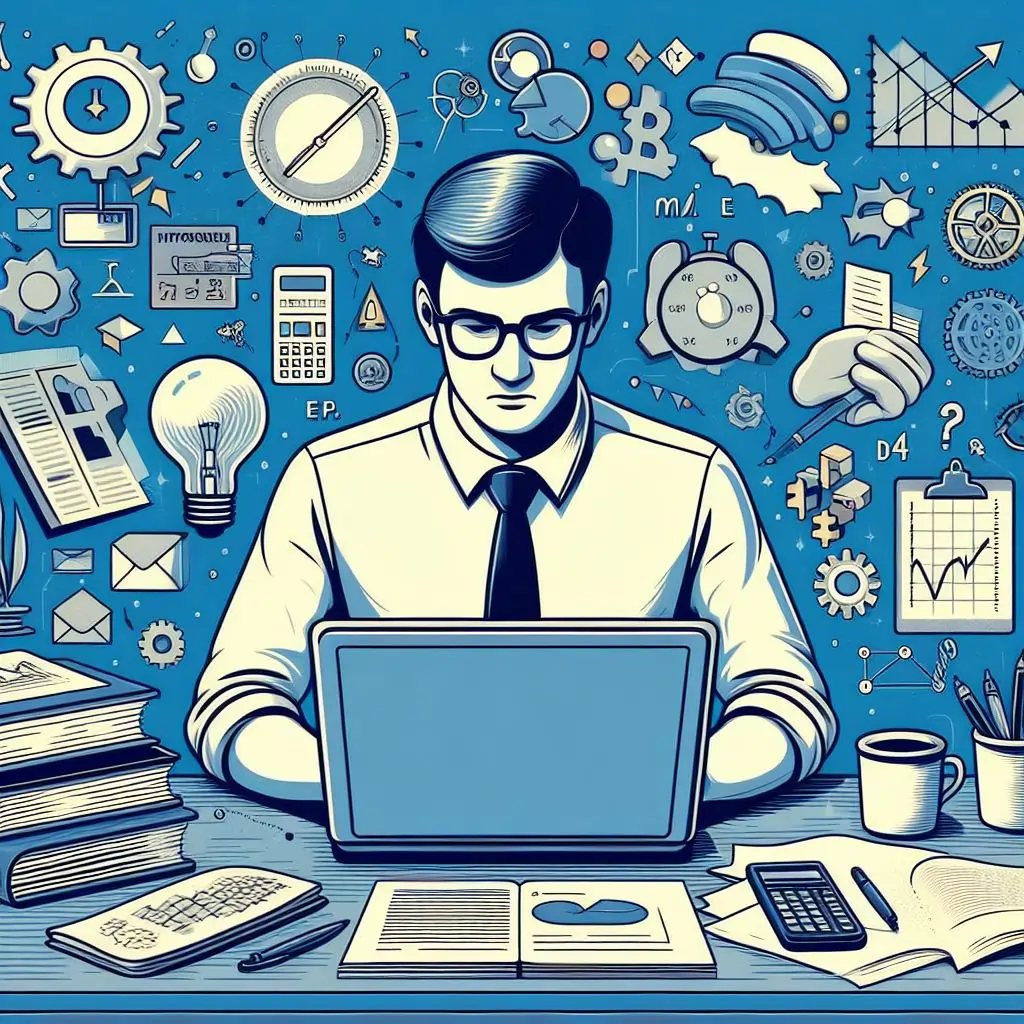
Whether unraveling the impact of government policies on economic growth or scrutinizing the intricate connections between consumer spending and GDP, students can wield hypothesis testing as a powerful tool to unveil the economic narratives hidden within the data. As this blog post unfolds, it serves as a comprehensive guide, offering students insights into the multifaceted realm of hypothesis testing in econometrics. By internalizing the nuances of each step—from defining variables and hypotheses to conducting tests and drawing conclusions—students can navigate their assignments with confidence, transforming their analyses into contributions that enrich the broader field of economics. In essence, hypothesis testing emerges not merely as an academic exercise but as a critical instrument that empowers students to unravel the complexities of economic relationships and make meaningful contributions to the ever-evolving tapestry of econometrics.
Understanding Hypothesis Testing in Econometrics
In the intricate domain of econometrics, hypothesis testing stands as a pivotal process, serving as the bedrock for making insightful inferences about population parameters from sample data. This methodological approach involves the systematic formulation of hypotheses concerning specific economic relationships or phenomena, followed by the rigorous application of statistical methods to scrutinize the validity of these propositions. The essence of hypothesis testing lies in its ability to distill complex economic theories into testable statements, allowing researchers to draw meaningful conclusions from empirical data. As econometricians grapple with questions about the impact of variables on economic outcomes or the validity of economic models, hypothesis testing emerges as the compass guiding them through the intricate maze of data analysis. This systematic methodology not only illuminates the relationships between economic variables but also provides a structured framework for assessing the robustness of economic theories in the face of empirical evidence. Consequently, understanding hypothesis testing in econometrics is tantamount to wielding a powerful analytical tool that empowers researchers to navigate the uncertainties inherent in economic phenomena, ensuring that the inferences drawn are not only theoretically grounded but also statistically sound.
Formulating Hypotheses
Before delving into the intricate world of hypothesis testing, it is imperative to grasp the art of formulating hypotheses accurately. In econometrics, hypotheses typically revolve around the relationships between variables. For instance, one might hypothesize that there exists a substantial relationship between the unemployment rate and inflation rate, setting the stage for a nuanced exploration of these economic indicators.
Null and Alternative Hypotheses
At the core of hypothesis testing lie two pivotal hypotheses—the null hypothesis (H0) and the alternative hypothesis (H1). The null hypothesis often embodies the status quo or the absence of an effect, while the alternative hypothesis posits a change or difference. Applying this to the unemployment and inflation scenario, the null hypothesis could assert that there is no significant relationship between the two, while the alternative hypothesis would counter that proposition.
Choosing the Significance Level
A critical aspect in hypothesis testing is the selection of the significance level, denoted as α, which serves as the threshold for rejecting the null hypothesis. Commonly adopted values for α include 0.05 or 5%, yet researchers possess the flexibility to opt for different levels based on contextual considerations and their desired level of confidence in the resulting conclusions.
Selecting a Test Statistic
The choice of a suitable test statistic hinges on the nature of the data and the specific hypothesis under scrutiny. Econometricians confront a plethora of options, including t-tests, F-tests, and chi-square tests, necessitating a judicious selection aligning with the intricacies of their research questions.
Collecting and Analyzing Data
With hypotheses formulated and a test statistic chosen, the next pivotal phase involves the collection and analysis of data. The utilization of econometric software such as R, Python, or statistical packages like STATA and SAS streamlines this process. The overarching goal is to discern whether the observed data furnishes adequate evidence to warrant the rejection of the null hypothesis.
Interpreting Results
Upon completing the hypothesis test, researchers confront the task of interpreting the results. If the p-value, denoting the probability, falls below the chosen significance level, the null hypothesis is discarded. This signifies compelling evidence in favor of the alternative hypothesis. Conversely, if the p-value surpasses the designated significance level, the null hypothesis remains unscathed. This interpretative step is pivotal, requiring researchers to synthesize statistical outcomes with economic intuition to draw meaningful and contextually relevant conclusions from their analyses.
Practical Application in University Assignments
The transition from theoretical understanding to practical application is paramount for students seeking excellence in their university assignments centered around hypothesis testing in econometrics. Armed with a foundation in the intricacies of formulating hypotheses, discerning between null and alternative hypotheses, and choosing significance levels and appropriate test statistics, students are poised to tackle real-world economic questions. Selecting relevant topics aligned with their coursework interests, such as analyzing the impact of government policies on economic growth or investigating the relationship between consumer spending and GDP, provides students with a meaningful context for their assignments. The process of defining variables and hypotheses becomes a critical precursor, as students articulate clear research questions, ensuring the subsequent collection and preparation of data is purposeful and conducive to robust analyses. Leveraging econometric software like R, Python, or statistical packages such as STATA and SAS, students can seamlessly execute hypothesis tests, transforming raw data into meaningful results. The interpretation of these results is the pinnacle of practical application, requiring students to not only understand statistical outcomes but also to contextualize their findings within the broader economic landscape. Thus, university assignments become a dynamic platform for students to showcase their adeptness in hypothesis testing, providing a conduit for the integration of theoretical knowledge with real-world problem-solving skills essential for success in the field of econometrics. In this practical realm, students not only demonstrate their mastery of the hypothesis testing process but also contribute meaningfully to the ongoing discourse in economics by applying their skills to unravel the complexities of economic relationships and phenomena.
Selecting Relevant Topics
Embarking on a successful journey in econometrics assignments begins with the pivotal task of selecting a relevant and intriguing topic. Aligning your choice with personal interests and coursework not only fosters engagement but also lays the foundation for a well-crafted assignment. Whether delving into the intricate analysis of government policies on economic growth or unraveling the complex relationship between consumer spending and GDP, the chosen topic sets the stage for a nuanced exploration that can significantly contribute to the field of econometrics.
Defining Variables and Hypotheses
The subsequent step involves the meticulous definition of variables and the formulation of hypotheses, which collectively serve as the bedrock for the entire hypothesis testing process. This critical phase requires clarity and precision, as researchers articulate the key elements they will study and craft hypotheses that align with their overarching research questions. By investing time in this foundational step, students ensure the coherence and validity of their subsequent analyses.
Data Collection and Preparation
With a well-defined research framework, the focus shifts to data collection and preparation. The quality of the analysis hinges on the accuracy, representativeness, and suitability of the gathered data for the chosen hypothesis testing method. Rigorous data cleaning and preparation are indispensable steps to guarantee the reliability of subsequent results, ensuring that the data reflects the nuances of the economic phenomena under investigation.
Choosing the Right Test Statistic
The nature of hypotheses and data dictates the choice of an appropriate test statistic, a decision that necessitates careful consideration and justification. In this section of the assignment, students demonstrate their understanding of various statistical methods and their ability to apply them judiciously to the specific context of their research. Clear and concise justifications enhance the credibility of the subsequent hypothesis testing process.
Conducting the Hypothesis Test
The utilization of econometric software becomes paramount as students transition to the actual hypothesis testing phase. Executing the test involves providing detailed steps, code, and output in the assignment, showcasing the rigor and transparency of the analysis. Additionally, researchers should discuss any assumptions made during the testing process, acknowledging the limitations and potential implications on the results.
Interpreting Results and Drawing Conclusions
The final stretch of the assignment entails the interpretation of results and the drawing of meaningful conclusions. This stage demands a synthesis of statistical outcomes with economic intuition, showcasing the researcher's comprehension of the broader implications of their findings. The discussion revolves around whether the null hypothesis is rejected, providing evidence in favor of the alternative hypothesis, or if it remains intact, influencing subsequent economic interpretations. In essence, this is the pinnacle where students exhibit their ability to translate statistical results into meaningful insights with profound economic implications.
To excel in hypothesis testing assignments in econometrics, consider the following tips:
- Understand the Economic Context: A foundational step involves gaining a thorough understanding of the economic context surrounding the research question. This understanding is crucial in formulating meaningful hypotheses that align with the intricacies of economic relationships and dynamics.
- Practice with Real Data:Theoretical knowledge alone is insufficient. To enhance practical skills in data collection, analysis, and interpretation, students must actively engage with real-world data. This hands-on experience not only reinforces theoretical concepts but also prepares students for the complexities of applying these concepts to actual economic scenarios.
- Seek Guidance: Hypothesis testing can be challenging, and seeking guidance is a proactive approach to overcoming difficulties. Professors, classmates, and online resources can provide valuable insights, clarifications, and additional resources to navigate through the intricacies of hypothesis testing.
- Stay Updated: The field of econometrics is dynamic, with continuous advancements and evolving techniques. To ensure long-term success, students should stay abreast of new developments and emerging methodologies in econometrics. This commitment to staying updated ensures that students' skills remain relevant and adaptable to the evolving landscape of economic research and analysis.
Conclusion
In conclusion, mastering the intricacies of hypothesis testing in econometrics is not merely an academic endeavor; it is a gateway to unlocking the analytical potential needed for success in university assignments and beyond. Selecting relevant topics, defining variables, meticulous data collection, and judicious interpretation of results constitute a comprehensive approach that transforms theoretical knowledge into practical skills. As students navigate the dynamic landscape of economic relationships, the application of hypothesis testing becomes a beacon, illuminating the path toward informed decision-making and insightful conclusions. With each assignment, students not only demonstrate their competence in statistical methodologies but also contribute meaningfully to the ongoing discourse in economics, solidifying their role as adept analysts in the ever-evolving field of econometrics.
Similar Blogs
At economicshomeworkhelper.com, we are dedicated to simplifying complex economic concepts and providing insightful analysis. Our blog is your go-to resource for expert tips, comprehensive guides, and the latest economic trends to help you excel in your studies and stay informed.