- Probability Theory in Econometrics
- Basic Concepts of Probability
- Probability Distributions
- Conditional Probability
- Distribution Theories in Econometrics
- Normal Distribution
- Student's t-Distribution
- Chi-Square Distribution
- Applications in Econometric Assignments
- Hypothesis Testing
- Regression Analysis
- Time Series Analysis
- Conclusion
Econometrics, an interdisciplinary field that seamlessly blends principles from economics, statistics, and mathematics, stands as a linchpin in the profound analysis of economic phenomena. Central to its core are the intricate threads of probability and distribution theories. In the dynamic landscape of econometrics, where uncertainty pervades every economic inquiry, probability theory emerges as the lodestar. Here, uncertainty becomes quantifiable, paving the way for a systematic exploration of economic uncertainties tied to human behavior, market dynamics, and external forces. From the foundational concepts of probability, where likelihoods are delineated on a scale from 0 to 1, to the nuanced understanding of probability distributions like the normal, binomial, and Poisson distributions, students embark on a journey of deciphering the likelihoods of diverse economic events. The journey continues with a meticulous exploration of conditional probability, a key instrument in modeling interrelated economic variables, reflecting the intricate web of dependencies that characterize economic relationships. Parallel to the orbit of probability theory, distribution theories come into play, steering the course of modeling economic variables. The normal distribution, a bedrock of econometrics, introduces students to the bell-shaped reality that encapsulates variables like income and stock returns. As assignments beckon, probability and distribution theories crystallize into practical tools. In the realm of hypothesis testing, students wield these tools to assess the veracity of conjectures surrounding economic phenomena, unraveling the statistical significance of their findings. Regression analysis, a sine qua non of econometrics, relies on these theories for validating assumptions, constructing confidence intervals, and deciphering the precision of regression coefficients. Time series analysis, a window into the temporal evolution of economic variables, finds its foundation in probability theories like autoregressive processes, propelling students into the heart of accurate forecasting. As this comprehensive guide unfolds, students are armed with more than theoretical insights—they possess a practical toolkit to solve their Econometrics homework and navigate the intricacies of econometric assignments. The synergy of probability and distribution theories becomes a compass guiding them through the complexities of modeling economic uncertainties, hypothesis testing, regression analysis, and time series exploration. Rooted in this understanding, students emerge equipped not only to tackle assignments with assurance but also to contribute meaningfully to the ongoing narrative in the field of economics. This guide, with its clarity and depth, serves as a beacon, illuminating the often challenging terrain of probability and distribution theories in econometrics, making it accessible, applicable, and empowering for students venturing into the rich tapestry of economic analysis.
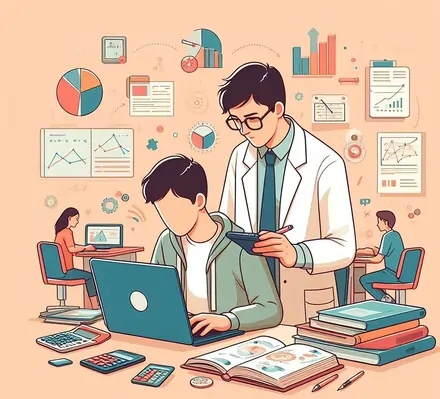
Probability Theory in Econometrics
Probability theory, a bedrock of econometrics, stands as the cornerstone providing a systematic and robust framework for modeling uncertainty—a pervasive element within the realm of economic analysis. The inherent uncertainty in econometrics stems from a myriad of factors ranging from the complexities of human behavior to the dynamism of market forces and the influence of external factors. It is within this intricate landscape that probability theory assumes paramount importance. By offering a means to quantify uncertainty, probability theory becomes an indispensable tool for econometricians, enabling them to navigate the intricacies of economic phenomena with precision and confidence. The very essence of econometrics lies in understanding and interpreting the uncertainty inherent in economic data, and probability theory serves as the compass that not only acknowledges this uncertainty but transforms it into a quantifiable entity. As econometricians grapple with the challenges posed by the unpredictable nature of economic systems, probability theory emerges as a guiding light, allowing for the formulation of robust models and the nuanced interpretation of data. Whether unraveling the uncertainties associated with human decision-making or deciphering the unpredictable shifts in market dynamics, probability theory, with its mathematical rigor and conceptual clarity, empowers econometricians to not only make sense of the complex economic landscape but also to make informed predictions and decisions based on a profound understanding of uncertainty. In essence, probability theory in econometrics transcends mere mathematical abstraction; it becomes the lens through which econometricians gain insights into the uncertainties that define and shape economic realities, making it an indispensable ally in the pursuit of rigorous and meaningful economic analysis.
Basic Concepts of Probability
Initiating the journey into probability theory, one encounters the foundational concepts that underpin the entire framework. At its essence, probability is a measure of the likelihood of an event occurring. In the multifaceted realm of econometrics, these events span a spectrum, from the success of a business venture to the probability of inflation surpassing a predetermined threshold. The gradation of probability occurs on a scale from 0 to 1, with 0 denoting impossibility, 1 representing certainty, and values in between offering a nuanced representation of varying degrees of likelihood. This foundational understanding provides econometricians with the tools to navigate uncertainty and quantify the likelihood of diverse economic events.
Probability Distributions
Building upon the basic concepts, probability distributions become the canvas on which the uncertainties of statistical experiments are painted. In econometrics, grasping the distribution of variables is pivotal. The arsenal of common probability distributions in this field includes the ubiquitous normal distribution, the versatile binomial distribution, and the discrete Poisson distribution. The selection of a specific distribution hinges on the nature of the data under scrutiny and the assumptions woven into the fabric of the econometric model. Here, probability distributions serve as a crucial bridge, linking theoretical concepts with the practical application of econometric models.
Conditional Probability
In the intricate tapestry of econometrics, where variables seldom exist in isolation, the concept of conditional probability takes center stage. This dimension measures the likelihood of an event occurring given the occurrence of another event, a fundamental aspect in modeling the complex interdependencies inherent in economic relationships. From forecasting market trends to understanding consumer behavior, conditional probability emerges as an instrumental tool, offering econometricians the ability to capture the nuanced dynamics where the outcome of one economic event is intricately tied to the occurrence of another. In essence, conditional probability becomes the thread that weaves a more intricate and realistic narrative of economic phenomena in the realm of econometrics.
Distribution Theories in Econometrics
Distribution theories stand as essential pillars in the realm of econometrics, serving as the guiding light for modeling the intricate behavior of economic variables. In the dynamic landscape of economic analysis, where data embodies the multifaceted nature of real-world phenomena, distribution theories offer a structured framework to comprehend the dispersion of data points and assess the probability of observing specific values. Central to this understanding is the recognition that economic variables seldom adhere to a uniform pattern; instead, they exhibit diverse behaviors and patterns. The application of distribution theories, therefore, becomes paramount in unraveling the inherent complexities of economic data. Whether exploring the variance in income levels, analyzing the distribution of stock returns, or assessing the spread of inflation rates, distribution theories provide the econometrician with a lens to interpret and quantify the inherent variability within economic variables. This interpretive framework extends beyond mere statistical abstraction; it becomes a powerful tool for econometricians to discern patterns, identify outliers, and make informed predictions about the likelihood of specific economic outcomes. As econometric assignments beckon, the mastery of distribution theories becomes indispensable for students navigating the intricacies of model construction, hypothesis testing, and data interpretation. The normal distribution, with its characteristic bell-shaped curve, becomes a bedrock for understanding the general tendencies of economic variables, while other distributions, such as the skewed distributions or heavy-tailed distributions, capture the nuances of specific economic phenomena. The application of these theories is not a mere technical exercise; it is an intellectual endeavor that empowers econometricians to distill meaningful insights from the often noisy and unpredictable world of economic data. In essence, distribution theories in econometrics transcend their mathematical formulations; they become the compass guiding researchers through the labyrinth of economic variability, allowing them to draw meaningful inferences, construct robust models, and contribute meaningfully to the understanding and prediction of economic phenomena.
Normal Distribution
The normal distribution, often referred to as the Gaussian distribution, stands as a fundamental concept in econometrics, permeating various facets of statistical analysis. Its significance lies in the observation that numerous economic variables, ranging from income levels to stock returns, conform to a bell-shaped distribution. This ubiquitous pattern forms the basis for understanding and modeling the behavior of these variables. Mastery of the properties of the normal distribution is crucial for econometricians engaged in hypothesis testing and the construction of confidence intervals. By delving into the nuances of the normal distribution, econometricians gain insights into the probabilities associated with different outcomes, allowing them to make informed decisions about the statistical significance of their findings. As a cornerstone of statistical theory, the normal distribution provides a solid foundation for conducting rigorous analyses in econometrics.
Student's t-Distribution
In the realm of econometrics, where researchers often contend with limited sample sizes, the student's t-distribution emerges as a reliable tool for accurate statistical inference. Small sample sizes can introduce increased uncertainty into the analysis, and the t-distribution accounts for this by providing a more nuanced representation of uncertainty. Understanding when to deploy the t-distribution becomes paramount for econometricians navigating the intricacies of small data sets. Whether estimating population parameters or conducting hypothesis tests, a judicious application of the t-distribution ensures that researchers draw robust and accurate inferences from limited data. As econometric assignments unfold, students must grasp the intricacies of the t-distribution, recognizing its role as a safeguard against the pitfalls associated with small sample sizes, thereby enhancing the accuracy and reliability of their analyses.
Chi-Square Distribution
In the mosaic of econometric tools, the chi-square distribution assumes a prominent role, particularly in the context of hypothesis testing and model validation. This distribution finds its application when testing relationships between categorical variables and evaluating the goodness of fit of econometric models. Mastery of the chi-square distribution is imperative for students immersed in assignments involving model validation. As econometricians seek to validate their models against real-world data, the chi-square distribution becomes a compass, guiding them through the assessment of model adequacy and identifying areas where adjustments may be necessary. Whether employed in contingency table analyses or assessing the fit of regression models, the chi-square distribution is a versatile and indispensable tool that empowers students to critically evaluate the validity of their econometric models.
Applications in Econometric Assignments
Applications in econometric assignments transcend theoretical concepts, demanding a seamless integration of probability and distribution theories into real-world economic analysis. As students delve into the intricate landscape of econometric assignments, these foundational theories become indispensable tools for hypothesis testing, regression analysis, and time series exploration. In the realm of hypothesis testing, probability concepts are wielded with precision, allowing students to assess the validity of conjectures surrounding economic phenomena. The significance of probability distributions comes to the forefront in regression analysis, where understanding the distribution of residuals is pivotal for validating the assumptions of the model. Armed with probability and distribution theories, students construct confidence intervals, unravel the precision of regression coefficients, and make informed decisions about the statistical significance of their findings. Time series analysis, a critical aspect of econometrics dealing with the temporal evolution of economic variables, relies on probability theories such as autoregressive processes to model temporal dependencies accurately. As assignments beckon, students navigate the complexities of economic data armed with a practical toolkit, applying probability and distribution theories to construct robust models and make informed predictions. The normal distribution, a stalwart in econometrics, becomes a beacon for understanding general tendencies, while specialized distributions cater to the nuances of specific economic phenomena. This application-oriented approach transforms abstract theories into powerful instruments for forecasting market trends, assessing the impact of economic policies, and unraveling the intricate dynamics of economic systems. Beyond statistical abstraction, probability and distribution theories in econometric assignments become a lens through which students interpret real-world economic phenomena, fostering a deeper understanding of the uncertainties that permeate economic data. In this dynamic interplay of theory and application, students not only unravel the intricacies of econometric assignments but also contribute meaningfully to the ongoing narrative in the field of economics. As they navigate the challenges posed by diverse datasets and complex economic relationships, the application of probability and distribution theories equips students with the analytical acumen to navigate the multifaceted landscape of economic analysis, ensuring that their contributions to the field are both rigorous and insightful.
Hypothesis Testing
Econometric assignments frequently immerse students in the realm of hypothesis testing, a pivotal exercise where the validity of conjectures about economic phenomena is scrutinized. At the heart of this analytical journey lie probability and distribution theories, serving as the bedrock for making informed decisions about the statistical significance of findings. Armed with these theoretical tools, students navigate the complexities of economic data, employing probability concepts to quantify uncertainty and draw robust conclusions. Whether assessing the impact of policy changes or examining the relationships between economic variables, probability and distribution theories empower students to critically evaluate hypotheses, ensuring their contributions to economic analysis are grounded in statistical rigor.
Regression Analysis
Central to the econometric toolkit, regression analysis relies heavily on the application of probability and distribution theories. As students engage in regression modeling, understanding the distribution of residuals takes precedence, acting as a compass for validating the assumptions underpinning the regression model. Probability concepts come to the fore, enabling students to construct confidence intervals that provide a measure of certainty around their estimates. Assessing the precision of regression coefficients becomes a nuanced exercise, where probability and distribution theories offer students the analytical tools to distinguish between significant and inconclusive results. In the intricate dance between theory and application, regression analysis emerges as a robust method, with probability and distribution theories guiding students through the maze of economic relationships and model complexities.
Time Series Analysis
The temporal evolution of economic variables introduces a unique set of challenges, met head-on through time series analysis in econometrics. Probability theories, particularly autoregressive processes, become invaluable in modeling the temporal dependencies woven into economic data. Students navigating time series assignments delve into the intricacies of probability distributions, understanding the nuances of variables evolving over time. This comprehension of distribution dynamics becomes fundamental in accurate forecasting, where probabilistic assessments are essential for predicting future economic trends. Time series analysis, anchored in probability and distribution theories, equips students with the tools to unravel the temporal complexities inherent in economic data, providing a lens through which they can discern patterns, forecast future trends, and contribute meaningfully to the predictive landscape of economic analysis.
Conclusion
In conclusion, a solid grasp of probability and distribution theories is indispensable for excelling in econometrics assignments. This guide has provided a simplified overview of these concepts, emphasizing their applications in real-world economic analysis. Armed with this knowledge, students can confidently navigate the complexities of econometric assignments, making informed decisions and contributing meaningfully to the field of economics.