- Understanding Endogeneity
- Endogeneity in Regression Models
- Motivation for Instrumental Variables
- Identifying and Choosing Instruments
- Relevance Condition
- Over-Identification Test
- Implementing Instrumental Variables Analysis
- Two-Stage Least Squares (2SLS)
- Interpretation of IV Results
- Potential Pitfalls and Solutions
- Endogeneity Concerns
- Instrument Selection
- Conclusion
Instrumental Variables (IV) analysis stands as a robust statistical method, finding extensive application in econometrics and various fields, acting as a beacon to illuminate the path through the intricate terrain of endogeneity issues and the pursuit of causal relationships between variables. As students embark on their journey into the multifaceted realm of econometrics, the comprehension of IV analysis becomes pivotal, forming a linchpin for effectively addressing the intricacies embedded in complex assignments and ambitious research projects. This comprehensive guide aims to dissect the fundamentals of Instrumental Variables Analysis, offering students a meticulous, step-by-step approach that serves as a navigational tool through the labyrinth of concepts and real-world applications. By unraveling the layers of IV analysis, this guide endeavors to empower students with the knowledge and skills necessary to not only grasp the theoretical underpinnings but also to proficiently apply these concepts to their academic endeavors. With a focus on clarity and accessibility, each section delves into critical aspects, from comprehending the insidious nature of endogeneity in regression models to the nuanced process of selecting and validating instruments. Motivating the need for instrumental variables, this guide elucidates the significance of external variables that are correlated with the endogenous variable but maintain a disassociation from the dependent variable, forming the bedrock for the entire analytical process. In the pursuit of instrument selection, the relevance condition takes center stage, compelling students to grapple with the intricacies of correlation, while the over-identification test emerges as the litmus test for the robustness and sufficiency of chosen instruments. Transitioning from theory to practice, the guide equips students with the tools to implement Instrumental Variables Analysis through the two-stage least squares (2SLS) method, fostering a hands-on understanding of this indispensable statistical technique. Beyond the technicalities, the guide acknowledges the potential pitfalls that students may encounter, emphasizing the importance of addressing endogeneity concerns and providing strategies for navigating the complexities of instrument selection. By encapsulating the essence of Instrumental Variables Analysis, this guide serves as an indispensable resource for students, unraveling the layers of complexity inherent in econometrics, and offering a clear roadmap for not only mastering the theoretical foundations but also applying them with confidence and precision in the pursuit of academic excellence. If you need assistance in solving your Econometrics homework, this guide provides a comprehensive and insightful approach to guide you through the process.
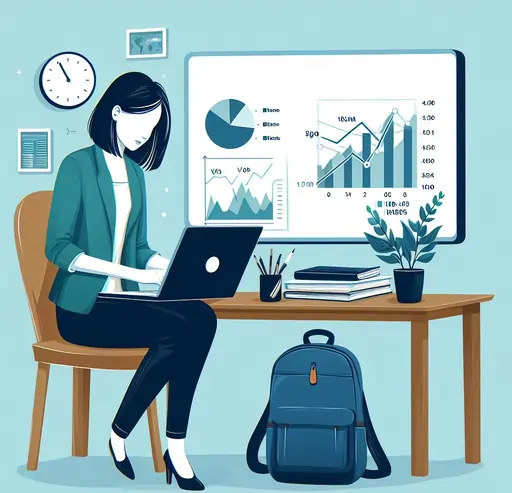
Understanding Endogeneity
In the intricate landscape of econometrics, Section 1, dedicated to understanding endogeneity, unveils a pervasive challenge that often complicates statistical analyses. Endogeneity emerges when an explanatory variable becomes correlated with the error term within a regression model, introducing a conundrum that can severely compromise the integrity of empirical findings. This correlation, if left unaddressed, manifests as biased and inefficient estimates, creating a formidable barrier to the establishment of causal relationships between variables. The gravity of this issue necessitates a foundational understanding for students venturing into the realm of econometrics. Before immersing themselves in the nuanced world of Instrumental Variables Analysis, students are urged to grapple with the intricacies of endogeneity and its far-reaching implications. This preparatory step serves as a crucial cornerstone, laying the groundwork for the comprehensive exploration of Instrumental Variables Analysis that follows in subsequent sections. By elucidating the challenges posed by endogeneity, Section 1 not only sheds light on a common hurdle in statistical analysis but also underscores the importance of equipping students with the necessary knowledge to navigate these challenges adeptly. In essence, the section acts as a gateway, compelling students to confront the subtleties of endogeneity head-on, ensuring a robust foundation for their subsequent engagement with the intricacies of econometric methodologies such as Instrumental Variables Analysis.
Endogeneity in Regression Models
We delve into the intricate realm of endogeneity in regression models, unraveling the subtleties of its manifestation and the consequential impact on parameter estimates. Endogeneity arises when an explanatory variable becomes correlated with the error term within a regression model, setting the stage for potential biases and inefficiencies in estimation. By elucidating this complex interplay, students gain a nuanced understanding of how endogeneity poses a formidable challenge to the integrity of statistical analyses. To enhance comprehension, illustrative examples are employed, providing tangible scenarios that underscore the practical implications of endogeneity, fostering a deeper grasp of its theoretical underpinnings.
Motivation for Instrumental Variables
The focus shifts towards the motivation behind Instrumental Variables Analysis as a potent remedy for endogeneity. Here, we elucidate the fundamental concept that sets Instrumental Variables Analysis apart – the introduction of an external variable, or instrument, that correlates with the endogenous variable. The instrument, however, maintains a crucial disassociation from the dependent variable. This subsection aims to convey the intrinsic need for such instruments in overcoming endogeneity challenges. By establishing this connection, students are guided towards a comprehensive understanding of the rationale behind employing instrumental variables, setting the stage for their exploration of the analytical technique. Emphasizing the importance of this motivation lays a solid foundation for students, ensuring they appreciate the purpose and relevance of Instrumental Variables Analysis in the broader context of econometrics.
Identifying and Choosing Instruments
In the expansive landscape of econometrics, It assumes a pivotal role, focusing on the meticulous process of identifying and selecting instruments – a critical step in the journey of Instrumental Variables (IV) analysis. The efficacy of IV analysis hinges on the judicious selection of instruments that satisfy stringent criteria, and this section serves as a beacon guiding students through this intricate task. As students delve into the complexities of this section, they encounter the cornerstone concept of the relevance condition. This condition stipulates that instruments must not only be correlated with the endogenous variable but also possess a distinct lack of direct correlation with the dependent variable. The intricacies of correlation and disassociation become paramount as students navigate the nuances of relevance conditions, and this section aims to demystify these concepts, ensuring a solid grasp of the principles that underpin the entire IV analysis process.
Navigating through the section, students are presented with the significance of a valid instrument's correlation with the endogenous variable – a correlation that is not merely coincidental but is intricately linked to the analytical task at hand. The gravity of this step becomes apparent as students comprehend the consequences of weak instruments, which can jeopardize the validity of the IV estimates. Through illustrative examples and practical scenarios, this section aims to equip students with the analytical acumen needed to identify instruments that meet the stringent relevance conditions. By delving into this process, students not only refine their theoretical understanding but also develop practical skills in instrument selection, laying the groundwork for their subsequent foray into the application of IV analysis. Thus, It serves as a comprehensive guide, preparing students to navigate the intricate landscape of selecting instruments, ensuring they emerge with the expertise required to conduct robust and meaningful Instrumental Variables Analysis in their academic pursuits.
Relevance Condition
It delves into the intricacies of the relevance condition, a pivotal concept in the realm of Instrumental Variables (IV) analysis. This condition demands that instruments chosen for the analysis must exhibit a meaningful correlation with the endogenous variable. Emphasizing the significance of this correlation, the subsection elucidates how the relevance condition is the bedrock upon which the validity of IV estimates rests. By establishing a clear connection between the instruments and the endogenous variable, students are guided to appreciate the nuanced dynamics that ensure the instrumental variable's efficacy. To bolster understanding, concrete examples are presented, illustrating how weak instruments can compromise the validity of IV estimates. Through these examples, students gain insight into the potential pitfalls of instrument selection, fortifying their ability to discern between robust and feeble instruments in their own analytical endeavors.
Over-Identification Test
Attention turns to the concept of over-identification tests, a critical tool in the toolkit of IV analysis. These tests serve as a mechanism to assess the validity of chosen instruments by determining if the set of instruments selected is both necessary and sufficient. Introducing students to this evaluative process, the subsection elucidates the rationale behind over-identification tests and their role in bolstering the robustness of IV analysis. By engaging with these tests, students gain a deeper understanding of how to scrutinize the instruments they have selected, ensuring that they not only meet the relevance condition but also pass the stringent test of over-identification. Through a comprehensive discussion, students are empowered to navigate the intricate terrain of instrument validation, honing their skills in selecting instruments that are not only correlated with the endogenous variable but also collectively constitute a valid and comprehensive set for the task at hand.
Implementing Instrumental Variables Analysis
In the unfolding narrative of instrumental prowess in econometrics, Section 3 emerges as a guiding light, ushering students into the practical realm of implementing Instrumental Variables Analysis (IV). Here, the focus pivots from theoretical underpinnings to the tangible application of IV analysis using statistical software, aiming to furnish students with a hands-on experience that transcends the boundaries of abstraction. This section becomes a virtual mentor, providing a step-by-step walkthrough of the pragmatic steps required to execute IV analysis seamlessly. By doing so, students are not only introduced to the theoretical foundations of IV analysis, as explored in earlier sections, but are also equipped with the practical skills necessary to navigate statistical software interfaces with confidence and precision. The power of this hands-on engagement lies in its ability to bridge the gap between conceptual understanding and practical execution, ensuring that students not only comprehend the intricacies of IV analysis but can also wield this statistical tool adeptly in their academic and research pursuits. By demystifying the process through a guided walkthrough, Section 3 empowers students to tackle complex econometric problems, fostering a sense of self-assurance as they navigate the intricate landscape of IV analysis. In essence, this section serves as a catalyst for transforming theoretical knowledge into practical competence, enriching the academic journey of students and preparing them for the challenges that await in the empirical realm of econometrics.
Two-Stage Least Squares (2SLS)
It plunges students into the intricacies of the Two-Stage Least Squares (2SLS) method, a cornerstone of Instrumental Variables Analysis (IV). This subsection unfolds the methodology, elucidating the sequential steps essential for estimating IV coefficients using the 2SLS approach. The two-stage process involves first predicting the endogenous variable using the chosen instruments and subsequently regressing the dependent variable on the predicted values. By breaking down this process, students gain a profound understanding of how the 2SLS method disentangles endogeneity issues, paving the way for robust causal inference. To solidify this theoretical understanding, a numerical example is meticulously presented, illustrating the step-by-step application of 2SLS. This exemplar not only reinforces the conceptual framework but also provides students with a tangible reference point for implementing 2SLS in their own empirical analyses.
Interpretation of IV Results
The focus shifts to the crucial task of interpreting results derived from Instrumental Variables Analysis. This subsection serves as a compass for students navigating the nuanced terrain of interpreting IV results, emphasizing the importance of discerning subtleties when compared to standard regression outcomes. Understanding the intricacies of IV results becomes paramount, given the unique considerations introduced by the instrumental variable approach. Students are guided through the process of deciphering coefficients and significance levels, fostering a nuanced comprehension of the causal relationships unveiled by the IV analysis. The subsection's emphasis on nuanced interpretation equips students with the discernment required to extract meaningful insights from their analyses, ensuring that they not only master the technical aspects of IV but also develop the interpretative skills vital for extracting real-world implications from their empirical findings. In essence, Subsection 3.2 serves as a beacon, guiding students to navigate the interpretative challenges inherent in IV analysis and encouraging a holistic understanding that transcends mere statistical outputs.
Potential Pitfalls and Solutions
As the academic journey through Instrumental Variables Analysis (IV) unfolds, It emerges as a beacon, shedding light on the potential pitfalls that students might encounter in their pursuit of econometric rigor and providing strategic insights to navigate these challenges adeptly. This section serves as a candid acknowledgment of the complexities inherent in IV analysis, recognizing that the path to robust causal inference is fraught with hurdles. By bringing these challenges to the forefront, students are forewarned and forearmed, equipped with the knowledge to anticipate and address these impediments proactively. The first step of this section involves the identification and understanding of common pitfalls in IV analysis, ranging from endogeneity concerns to issues related to instrument selection. By articulating these challenges, students are encouraged to develop a keen awareness of the potential pitfalls that may lurk in their empirical endeavors. Subsequently, Section 4 becomes a repository of solutions, offering a toolkit of strategies to fortify the analytical process. Whether addressing endogeneity through rigorous diagnostic checks or navigating the intricacies of selecting instruments that meet relevance conditions, the section acts as a guiding compass. By intertwining theoretical insights with practical solutions, this section aims not only to educate students on the potential pitfalls but also to empower them with the skills to surmount these challenges effectively. In essence, Section 4 encapsulates the resilience-building phase of the academic journey, recognizing that true mastery of Instrumental Variables Analysis extends beyond theoretical comprehension to the adept navigation of real-world complexities. Through this comprehensive guide, students are not only prepared to face the challenges inherent in IV analysis but also equipped with a repertoire of strategies to transform obstacles into opportunities for intellectual growth and methodological refinement.
Endogeneity Concerns
In the detailed exploration of challenges within Instrumental Variables Analysis (IV), Subsection 4.1 delves into the pervasive issue of endogeneity concerns. Here, the discussion centers on strategies for detecting endogeneity, acknowledging its potential to undermine the validity of IV estimates. The subsection emphasizes the pivotal role of robustness checks, elucidating their importance in fortifying the reliability of IV estimates. By systematically guiding students through various diagnostic tools and statistical tests, it equips them with the arsenal needed to identify and address endogeneity concerns. Recognizing the dynamic nature of empirical research, It becomes a compass for students, steering them toward a comprehensive understanding of the multifaceted strategies required to navigate the intricate terrain of endogeneity.
Instrument Selection
The spotlight shifts to the intricate task of instrument selection—a critical phase in the IV analysis process. The subsection not only acknowledges the challenges inherent in this step but also provides a compass for students to navigate these challenges with precision. By offering guidance on instrument selection, including the utilization of theoretical reasoning and sensitivity analyses, students are equipped with a strategic toolkit. Theoretical reasoning involves a thoughtful exploration of the relationships between instruments, endogenous variables, and the dependent variable, ensuring a grounded and well-informed selection process. Simultaneously, sensitivity analyses become a key component, enabling students to assess the robustness of their instrument choices across a spectrum of scenarios. Subsection 4.2 transforms the complexities of instrument selection into a structured and methodical process, empowering students to approach this critical aspect of IV analysis with confidence and precision. In essence, these subsections converge to form a comprehensive guide, ensuring that students not only recognize the potential pitfalls within IV analysis but also develop the analytical acumen to effectively mitigate these challenges in their academic and research endeavors.
Conclusion
In conclusion, Instrumental Variables Analysis stands as a crucial asset for students grappling with the intricacies of econometrics and confronting assignments riddled with endogeneity challenges. Proficiency in this analytical tool, coupled with a grasp of fundamental concepts and adept instrument selection, empowers students to refine their quantitative skills. By applying Instrumental Variables Analysis accurately, students not only surmount statistical hurdles but also contribute substantively to the landscape of rigorous empirical research within their academic pursuits. This analytical prowess becomes a catalyst for fostering a deeper understanding of economic phenomena and enhancing the precision and reliability of their quantitative analyses.