Claim Your Discount Today!
Unlock a fantastic deal at www.economicshomeworkhelper.com! Enjoy an exclusive 10% discount on all Economics homework this Spring Semester with the code EHHrSPRING10. Our expert team is ready to support you, ensuring top-quality assistance at the best price. Don’t miss this limited-time opportunity to excel in your studies while saving money. Use the code at checkout and secure expert help for your Economics homework today!
We Accept
- The Concept of Causality
- Definition and Importance
- Types of Causality
- Approaches to Establishing Causality
- Experimental Studies
- Observational Studies
- Causal Inference Methods
- Granger Causality
- Difference-in-Differences (DiD)
- Instrumental Variables (IV)
- Challenges in Causal Inference
- Endogeneity
- Selection Bias
- Case Studies and Real-World Applications
- Impact of Minimum Wage on Employment
- Effects of Education on Economic Growth
- Practical Tips for Causal Inference Homework
- Clearly Define Variables
- Choose Appropriate Methods
- Assess Assumptions
- Conclusion
In the dynamic realm of econometrics, a captivating fusion of economic theory, mathematics, and statistics converges to unravel and quantify the intricate web of economic relationships. Central to this discipline is the pivotal concept of causality, delving into the nuanced cause-and-effect relationships that underpin diverse economic variables. For students navigating the complex landscape of econometrics, a profound grasp of causality is indispensable, serving as the bedrock for crafting meaningful inferences and accurate predictions. This comprehensive guide endeavors to be a beacon of clarity and insight, offering students a profound understanding of causality in econometrics and arming them with the essential knowledge and skills needed to not only navigate their academic homework but also to embark on more substantial projects in the field. As students delve into the intricacies of econometrics, this guide serves as a compass, illuminating the path toward a nuanced comprehension of causality, a skill set that is paramount for those seeking to excel in the multifaceted world of economic analysis. By unraveling the layers of causality, students are empowered to not only interpret economic phenomena but also to contribute meaningfully to the ongoing discourse in the field. The guide addresses the varied approaches to establishing causality, from experimental studies that employ controlled environments to observational studies that grapple with the complexities of real-world data. It navigates the landscape of causal inference methods, exploring tools such as Granger Causality, Difference-in-Differences, and Instrumental Variables, each a valuable instrument in the econometric toolkit. If you find yourself in need of assistance with your econometrics homework, this guide is tailored to provide the support and insights required for success in your academic pursuits.
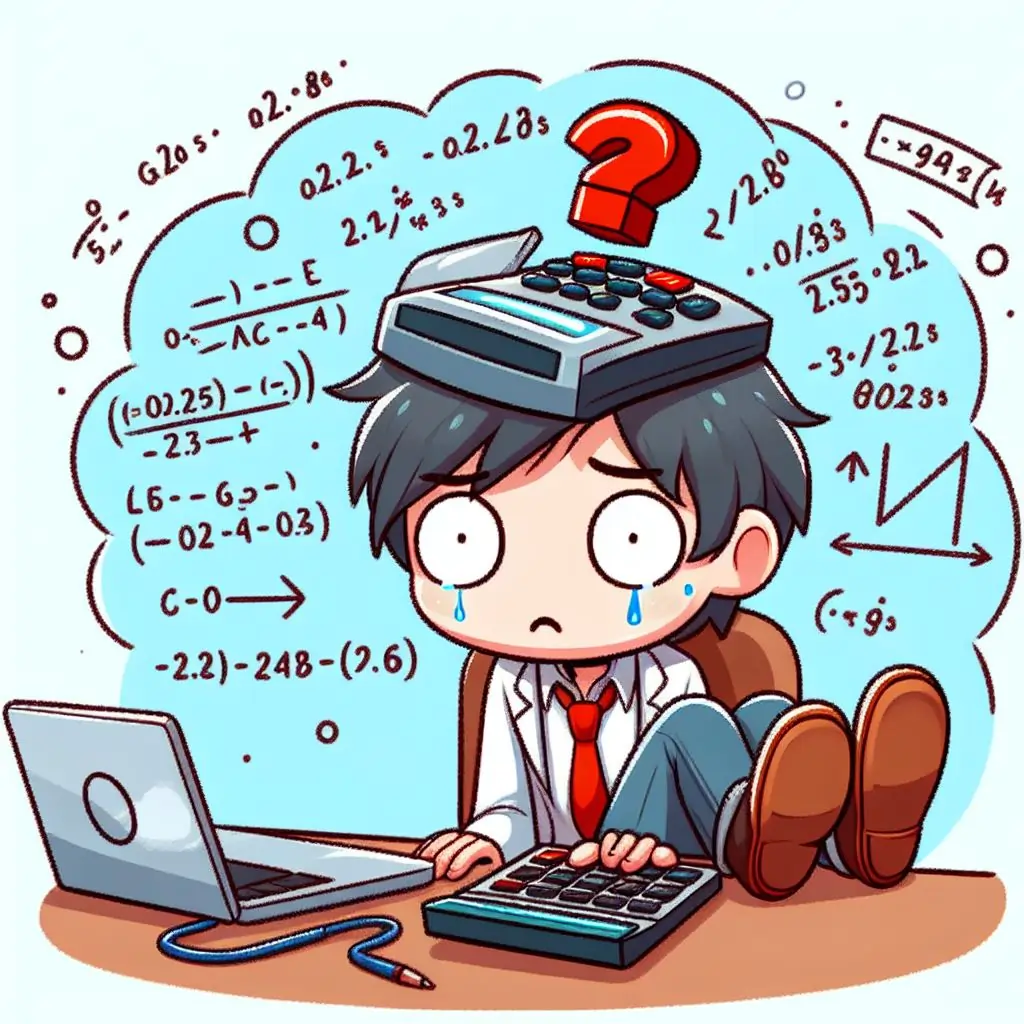
The narrative unfolds to illuminate the challenges inherent in causal inference, tackling issues like endogeneity and selection bias that often lurk beneath the surface of seemingly straightforward analyses. Real-world case studies further enrich the exploration, demonstrating the application of these concepts in practical scenarios, such as assessing the impact of minimum wage changes on employment levels or examining the intricate relationship between education and economic growth. Practical tips, seamlessly woven into the fabric of the guide, provide students with actionable advice, urging them to define variables with precision, select appropriate methods judiciously, and critically assess the assumptions that underlie their analyses. As the guide draws to a close, the overarching message is clear – mastery of causality in econometrics is not merely an academic pursuit but a gateway to becoming a discerning analyst capable of contributing substantively to the ongoing economic discourse. In the crucible of econometrics, this comprehensive guide stands as an indispensable resource, beckoning students to navigate the intricacies of causality with confidence and competence, empowering them to emerge not just as adept Homework solvers but as future contributors to the evolving landscape of economic knowledge.
The Concept of Causality
The concept of causality, central to the discipline of econometrics, serves as the keystone in unraveling the intricate relationships between economic variables. In its essence, causality delves into the cause-and-effect dynamics, seeking to understand the direct or indirect influence one variable has on another. This foundational idea is paramount for students navigating the complex landscape of econometrics, as it forms the bedrock for constructing meaningful analyses and deriving actionable insights. The understanding that correlation does not imply causation is a crucial starting point, prompting students to discern and substantiate causal relationships through rigorous methodologies. Within this conceptual framework, various types of causality emerge, such as direct and indirect causality, each contributing nuances to the understanding of how economic variables interconnect. As students embark on comprehending causality, they encounter the multifaceted nature of this concept, recognizing that establishing causation requires careful consideration of temporal sequences, logical reasoning, and statistical rigor. This section of the comprehensive guide provides a scaffolding for students to explore the layers of causality, laying the groundwork for subsequent discussions on experimental and observational studies, causal inference methods, and the challenges inherent in deciphering causal relationships in econometrics. By comprehensively grasping the concept of causality, students are equipped with a robust analytical framework, enabling them to dissect economic phenomena, make informed predictions, and contribute substantively to the field of econometrics. In essence, "The Concept of Causality" serves as the gateway to unlocking the deeper realms of econometric analysis, beckoning students to embark on a journey that transcends mere correlation, guiding them towards a nuanced understanding of the intricate web of cause-and-effect relationships that define the economic landscape.
Definition and Importance
Causality, within the realm of econometrics, constitutes the intricate relationship between two variables, wherein a modification in one variable precipitates a corresponding change in another. The significance of establishing causality is paramount as it serves as the linchpin for economists when formulating well-informed policy recommendations and predictions. An essential caveat in this exploration is the acknowledgment that correlation does not inherently imply causation. Navigating this distinction constitutes a fundamental aspect of econometric analysis, demanding a meticulous approach to disentangle the web of interrelated variables and discern the true causal pathways that underpin economic phenomena.
Types of Causality
Delving deeper into the fabric of causality, one encounters various typologies that elucidate the dynamics of cause-and-effect relationships:
- Direct Causality: This manifests when a discernible alteration in one variable precipitates an immediate and observable change in another.
- Indirect Causality: This complexity unfolds as a series of events, wherein alterations in one or more variables collectively contribute to subsequent changes in the target variable.
Appreciating and comprehending these distinctions proves to be pivotal when engaging in the intricate task of designing and interpreting econometric models. The nuanced understanding of direct and indirect causality equips economists and students alike with the analytical tools necessary to unravel the complexities inherent in economic relationships, facilitating more accurate and insightful modeling and forecasting.
Approaches to Establishing Causality
Navigating the multifaceted terrain of econometrics involves a critical exploration of diverse approaches aimed at establishing causality, a cornerstone in understanding the intricate relationships between economic variables. 2.1 Experimental Studies, characterized by controlled experiments where researchers manipulate one variable to observe its impact on another, stand as a robust methodological avenue. The gold standard in this domain is the Randomized Controlled Trial (RCT), providing a high level of control over extraneous factors. However, challenges such as ethical constraints and practical limitations often accompany experimental studies, prompting the consideration of alternative methodologies. 2.2 Observational Studies, an alternative trajectory, delve into naturally occurring data without external manipulation. While observational studies are more reflective of real-world conditions, they grapple with the complexity of confounding variables that may obscure causality. Within this context, techniques such as instrumental variables and matching methods emerge as valuable tools, helping to address endogeneity concerns and bolster the validity of causal inferences. As students immerse themselves in these approaches, they encounter the inherent trade-offs and complexities, recognizing the need for methodological diversity to suit the nature of the research question at hand. The ability to discern when experimental or observational methods are most appropriate becomes a critical skill, guiding students toward more robust and reliable causal inferences.
This exploration extends to 2.3 Causal Inference Methods, where techniques such as Granger Causality, Difference-in-Differences (DiD), and Instrumental Variables (IV) take center stage. Granger Causality, grounded in time-series analysis, investigates whether past values of one variable predict future values of another, providing insights into dynamic relationships. DiD, a quasi-experimental method, facilitates causal inference by comparing changes in outcomes between a treatment group and a control group over time. Instrumental Variables, on the other hand, address endogeneity issues by identifying variables correlated with the independent variable of interest but not directly linked to the dependent variable. Understanding the nuances of these methods equips students with a diverse toolkit, enabling them to adeptly navigate the intricacies of causal inference across varied research contexts. As students embark on Homework exploring causality in econometrics, this comprehensive guide seeks to empower them with the knowledge and analytical acumen needed to select, apply, and critically evaluate these approaches, fostering a deeper understanding of causality's elusive but essential nature in economic analysis.
Experimental Studies
Delving into the realm of experimental studies unveils a methodological approach where researchers meticulously manipulate one variable to discern its impact on another. At the forefront of experimental methodologies, Randomized Controlled Trials (RCTs) emerge as a prevalent tool, affording researchers a high degree of control over extraneous factors. This section of the comprehensive guide embarks on an exploration into the intricacies of experimental studies in econometrics, shedding light on their design principles and the challenges inherent in maintaining controlled environments. The discussion spans ethical considerations, practical limitations, and the nuanced application of RCTs, providing students with a comprehensive understanding of the strengths and limitations associated with this experimental approach.
Observational Studies
Contrasting the controlled environments of experimental studies, observational studies immerse themselves in naturally occurring data without external manipulation. While these studies offer a glimpse into real-world conditions, the quest to establish causality encounters heightened challenges due to potential confounding variables. In this context, techniques such as instrumental variables and matching methods come to the fore, offering methodological strategies to grapple with the complexities inherent in observational settings. This section of the guide navigates through the intricacies of observational studies, unveiling the analytical tools employed to enhance the validity of causal inferences. As students traverse this terrain, they gain insights into the art of disentangling causal relationships from the myriad factors that characterize real-world data, preparing them for the nuanced and challenging landscape of econometric analysis.
Causal Inference Methods
The exploration of causality in econometrics extends to a critical examination of various methods designed to disentangle the complex web of cause-and-effect relationships. 3.1 Granger Causality, a method named after Clive Granger, probes into the temporal dimension of causation within time-series analysis. It scrutinizes whether past values of one variable serve as predictors for future values of another, offering valuable insights into dynamic relationships. This section unravels the intricacies of Granger Causality, shedding light on its applications, strengths, and limitations, providing students with a profound understanding of its role in time-series econometrics. Complementing this is 3.2 Difference-in-Differences (DiD), a quasi-experimental method instrumental in comparing changes in outcomes between a treatment group and a control group over time. This technique, detailed within this section, stands as a powerful tool for causal inference in scenarios where randomized experiments are impractical. 3.3 Instrumental Variables (IV) emerges as a pivotal strategy to contend with endogeneity challenges by identifying variables correlated with the independent variable of interest but not directly influencing the dependent variable. As students traverse this comprehensive guide, they gain a nuanced comprehension of these causal inference methods, recognizing their applicability and limitations in diverse econometric scenarios. The guide encourages students to critically evaluate assumptions, understand the intricacies of each method, and apply them judiciously to derive robust causal inferences. By demystifying the complexities of Granger Causality, Difference-in-Differences, and Instrumental Variables, this guide equips students with a diverse analytical toolkit, empowering them to navigate the challenges posed by real-world data and contributing meaningfully to the field of econometrics. As they grapple with the subtleties of these methods, students embark on a journey of becoming discerning analysts capable of unraveling the causal threads that weave through the intricate fabric of economic relationships, reinforcing the fundamental role of causal inference methods in shaping rigorous and insightful econometric analyses.
Granger Causality
The terrain of econometrics introduces us to the Granger Causality method, an analytical approach named after Clive Granger. This method intricately explores the temporal dimensions of causation within time-series analysis, probing whether past values of one variable serve as predictive indicators for future values of another. Its widespread application in time-series analysis renders Granger causality a valuable tool, particularly adept at unraveling dynamic relationships between economic variables. As students delve into the nuances of this method, they gain a profound understanding of its theoretical underpinnings, practical applications, and the crucial role it plays in deciphering the intricate interplay of economic phenomena over time.
Difference-in-Differences (DiD)
In the realm of quasi-experimental methods, Difference-in-Differences (DiD) emerges as a consequential tool for causal inference. This method facilitates the comparison of changes in outcomes between a treatment group and a control group over time. Its value becomes pronounced in scenarios where the feasibility of randomized experiments is compromised, allowing researchers to estimate causal effects in observational settings. This section of the guide meticulously dissects the intricacies of DiD, exploring its conceptual foundations, applications, and the nuanced considerations that underlie its effective deployment in diverse econometric contexts.
Instrumental Variables (IV)
Instrumental Variables (IV) methods step into the forefront to address the perennial challenge of endogeneity in econometric analyses. By identifying variables that are correlated with the independent variable of interest but hold no direct influence over the dependent variable, IV methods offer a strategic approach to disentangle complex causal relationships. This section elucidates the assumptions and limitations inherent in instrumental variables, guiding students through the delicate balance required to employ this method effectively. As students navigate the landscape of instrumental variables, they not only gain a deeper understanding of the method itself but also cultivate the critical skills necessary for accurate and nuanced causal inference in the dynamic field of econometrics.
Challenges in Causal Inference
Navigating the landscape of causal inference in econometrics reveals a terrain marked by intricacies and challenges that demand careful consideration. The process of establishing causality is not without its pitfalls, and understanding and mitigating these challenges are essential for researchers and students alike. In this section, we delve into the complexities that often accompany causal inference, recognizing that the endeavor to unveil cause-and-effect relationships in economics is far from straightforward. As students grapple with these challenges, they confront the inherent intricacies of designing econometric models that can robustly capture the essence of causal relationships while navigating the potential pitfalls that may compromise the validity of their analyses. The challenges presented in this section are not mere obstacles; rather, they are opportunities for students to hone their analytical skills, develop a nuanced understanding of the limitations of various methodologies, and cultivate a discerning approach to causal inference in the ever-evolving field of econometrics. As researchers confront and overcome these challenges, they contribute not only to the advancement of their own academic pursuits but also to the broader discourse in economics, reinforcing the importance of methodological rigor and thoughtful consideration when unraveling the complex tapestry of cause-and-effect relationships in the economic realm.
Endogeneity
The landscape of causal inference in econometrics is intricately woven with challenges, and among them, endogeneity looms large. This phenomenon materializes when the independent variable becomes correlated with the error term, injecting bias into estimates and jeopardizing the validity of causal inferences. The critical juncture where endogeneity emerges demands meticulous attention, as failure to recognize and address it can lead to erroneous conclusions and misguided policy recommendations. Consequently, recognizing and untangling endogeneity becomes a linchpin in the quest for establishing causality in econometric models. This section of the guide meticulously dissects the complexities of endogeneity, delving into its conceptual foundations, methodological ramifications, and the arsenal of tools available to researchers to navigate this pervasive challenge. By equipping students with the knowledge and analytical skills to recognize and mitigate endogeneity, the guide empowers them to traverse the intricate landscape of econometric analysis with precision and confidence.
Selection Bias
In the tapestry of challenges inherent in causal inference, selection bias emerges as a prominent thread, introducing distortion when the sample fails to represent the broader population accurately. This bias skews results and poses a significant threat to the validity of causal inferences. Within this context, techniques like propensity score matching come to the fore as invaluable tools for mitigating selection bias. Propensity score matching aids in aligning the observed samples with the larger population, ensuring that the inferences drawn are not marred by systematic distortions. As students navigate the nuanced contours of selection bias, they delve into the methodological intricacies of propensity score matching, understanding its applications and limitations. Armed with this knowledge, students are better equipped to address selection bias in their econometric analyses, laying the groundwork for more accurate and reliable causal inferences in the realm of economic research.
Case Studies and Real-World Applications
In the pursuit of a comprehensive understanding of causality in econometrics, the integration of case studies and real-world applications stands as a pivotal bridge between theory and practice. This section not only enriches the theoretical foundation established earlier but also provides students with tangible examples that bring abstract concepts to life. Through the lens of case studies, students gain insight into the practical application of causal inference methods and the nuanced considerations involved in real-world economic scenarios. Consider exploring a case study that investigates the impact of minimum wage changes on employment levels, offering students an opportunity to apply and critically evaluate causal inference methods in the context of policy-relevant questions. Furthermore, an examination of the effects of education on economic growth can illuminate the broader implications of understanding causality. By immersing themselves in these real-world applications, students cultivate a more profound appreciation for the complexities of econometric analysis and the transformative potential of robust causal inference. The interplay between theory and application in this section serves as a cornerstone for students to bridge the gap between classroom learning and the practical challenges they will encounter in the dynamic landscape of economic research. As they engage with case studies and real-world applications, students are not merely passive recipients of knowledge; they become active participants, applying their analytical skills to unravel the intricacies of causality in authentic economic contexts. This integrative approach fosters a deeper comprehension of how causal inference methods contribute to informed decision-making and policy recommendations, solidifying the connection between the theoretical underpinnings of econometrics and its real-world impact.
Impact of Minimum Wage on Employment
Embarking on an exploration of the causal relationship between changes in minimum wage and employment levels, this case study stands as a beacon of practical application in the realm of econometrics. The scrutiny of minimum wage policies and their repercussions on employment is a pressing concern in economic discourse. By leveraging econometric methods to dissect this relationship, students gain a firsthand understanding of the complexities inherent in real-world scenarios. The practical example not only illustrates the application of causal inference methods but also underscores their transformative potential in informing policy decisions and shaping economic outcomes. As students engage with this case study, they unravel the intricate dance between economic variables, honing their analytical skills in the dynamic context of labor market policies.
Effects of Education on Economic Growth
Transitioning to a broader canvas, the case study examining the causal link between education and economic growth provides students with a panoramic view of the far-reaching implications of causality in econometrics. As students delve into the intricacies of this study, they uncover the profound interplay between education and economic prosperity. Analyzing the causal links between these variables not only showcases the importance of well-designed studies in informing policy decisions but also illuminates the pivotal role of education in fostering economic growth. This case study serves as a testament to the multifaceted impact of causal inference methods, emphasizing their significance in shaping the trajectory of nations and societies. By dissecting this causal link, students gain a nuanced understanding of the broader implications of their analytical endeavors, reinforcing the transformative potential of econometrics in driving informed and impactful policy decisions.
Practical Tips for Causal Inference Homework
As students venture into the realm of causal inference Homework in econometrics, a set of practical tips emerges as an invaluable compass, guiding them through the intricacies of designing, executing, and interpreting analyses. 6.1 Clearly Define Variables: Precision in variable definition is the cornerstone of any econometric analysis. Clearly articulate the variables under investigation, their relationships, and the expected outcomes to lay a robust foundation for your Homework. Ambiguity in variable definitions can lead to confusion and undermine the credibility of your analysis. 6.2 Choose Appropriate Methods: The choice of econometric methods should align with the nature of your data and research question. Justify your selection based on the characteristics of the variables, the study design, and the overarching goals of your Homework. A thoughtful and well-justified methodological approach enhances the robustness of your causal inference. 6.3 Assess Assumptions: Every econometric method is underpinned by a set of assumptions. Critically evaluate and clearly state these assumptions in your Homework. Recognizing and addressing assumptions head-on contributes to the transparency and reliability of your analysis. Acknowledging potential limitations demonstrates a nuanced understanding of the methodological framework. By adhering to these practical tips, students not only enhance the clarity and rigor of their causal inference homework but also cultivate a set of skills that extends beyond the specific task at hand. These tips foster a disciplined approach to econometric analysis, instilling in students the importance of precision, thoughtful methodology selection, and critical evaluation of assumptions. As students apply these practical tips, they embark on a journey of not only meeting the immediate demands of their homework but also cultivating a skill set that positions them as discerning analysts capable of navigating the complex landscape of causal inference in econometrics.
Clearly Define Variables
At the forefront of proficient causal inference in econometrics lies the imperative to precisely define variables. In the crucible of your homework, clarity in articulating the variables under scrutiny, their interrelationships, and anticipated outcomes serves as the bedrock for a robust analytical foundation. Ambiguity in variable definitions can introduce uncertainty, potentially leading to inaccurate conclusions. Therefore, a meticulous approach to defining variables becomes paramount, ensuring that the analytical framework is anchored in a clear and unambiguous understanding of the economic phenomena at hand. As students navigate their Homework, this emphasis on precise variable definition emerges as a fundamental guideline, elevating the quality and accuracy of their causal inference analyses.
Choose Appropriate Methods
The judicious selection of econometric methods is a strategic choice that significantly influences the integrity of causal inference. In the tapestry of your homework, aligning chosen methods with the inherent nature of your data and the specific contours of your research question is essential. Rigorously justify the selection of your chosen methods, considering the characteristics of the variables, the intricacies of the study design, and the overarching objectives of your Homework. This methodological alignment ensures that the chosen tools are not only suitable but also optimize the analytical process. By adhering to this principle, students not only exhibit a discerning approach to method selection but also enhance the overall coherence and validity of their causal inference homework.
Assess Assumptions
The underpinning assumptions of chosen econometric methods wield significant influence over the credibility of your analysis. In the terrain of homework, transparency is key – clearly state and critically evaluate these assumptions. By confronting and assessing the assumptions head-on, students manifest a sophisticated understanding of the methodological framework, establishing a foundation of trustworthiness. Furthermore, acknowledging potential limitations inherent in these assumptions adds a layer of nuance to the analysis, enhancing the overall credibility of the causal inference. As students engage with this aspect of their homework, they cultivate a rigorous and self-reflective approach, positioning themselves as meticulous analysts capable of navigating the intricacies of econometric analysis with both precision and insight.
Conclusion
The intricate landscape of causal inference in econometrics presents formidable challenges that demand a nuanced and sophisticated approach. As we navigate the complexities inherent in economic data, it becomes increasingly clear that achieving robust analyses requires a commitment to methodological rigor, a deep understanding of underlying assumptions, and a continual refinement of statistical tools. The dynamic interplay of various factors, endogeneity concerns, and unobservable variables underscores the need for researchers to employ innovative techniques and leverage advances in statistical methodologies.
Addressing the challenges in causal inference is not a one-size-fits-all endeavor; rather, it necessitates a contextual understanding of the specific economic phenomena under investigation. Transparency in modeling decisions, careful consideration of potential biases, and robustness checks are crucial elements in ensuring the reliability of causal inferences. Additionally, embracing interdisciplinary collaboration and learning from the wealth of diverse perspectives can contribute to the development of more resilient econometric models.
As we continue to grapple with the intricacies of causal inference, acknowledging the limitations and uncertainties inherent in econometric analyses is paramount. A commitment to open dialogue, ongoing refinement of methodologies, and a dedication to intellectual humility will undoubtedly enhance our ability to draw meaningful and reliable conclusions in the ever-evolving field of econometrics. By meeting these challenges head-on, researchers can contribute to the advancement of knowledge and foster a more accurate and nuanced understanding of the complex economic systems that shape our world.
You Might Also Like
Delve into a trove of knowledge and insights with our captivating blogs. From expert tips to industry trends and innovative solutions, discover fresh perspectives on topics that resonate with you. Stay informed, inspired, and empowered as you explore our latest posts.